SQL Materialized Views
Operate ML in Production
with a Streaming Database
Materialize is a database that helps teams solve data latency, quality, monitoring challenges when operating ML and online feature-serving at scale.
We decided to look into Materialize to handle personalization and feature-serving in real-time, and by that evening we were up and running.
Tom Cooper Head of Data, Superscript
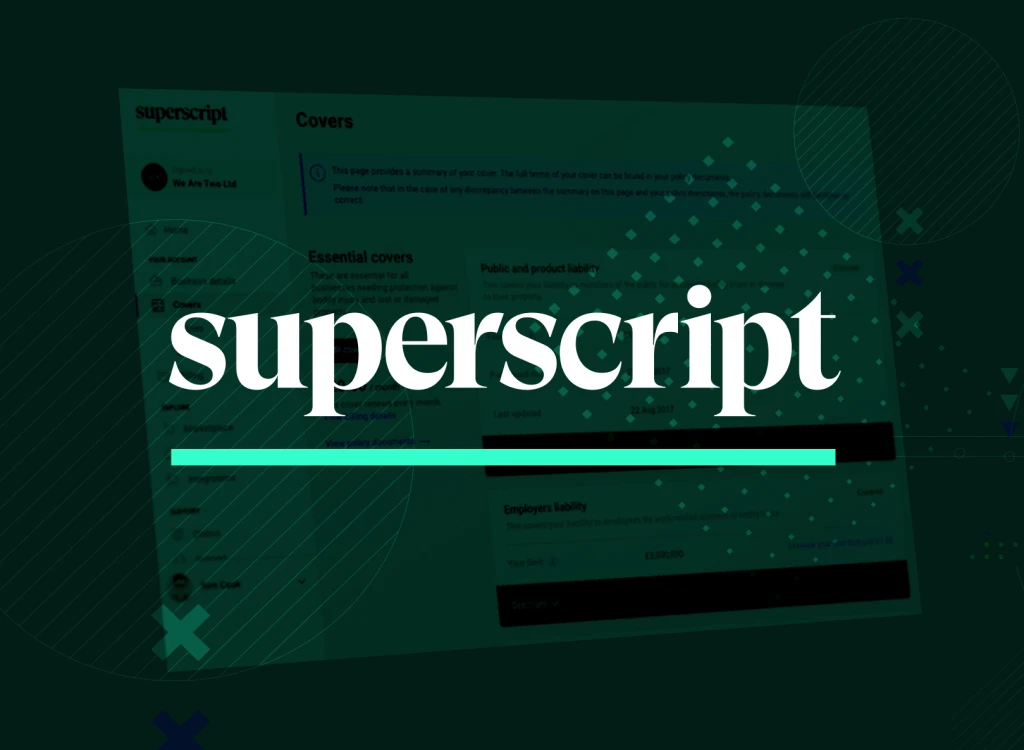
Put Data to Work in Machine Learning Use Cases
Real-time online feature serving
Use Materialize to complement your offline feature store, which is built primarily to store and access historical feature data. Build real-time predictions with millisecond latency reads and high throughput writes with Materialize.
Operate on multiple data sources
Materialize supports cross-stream and multi-way joins, without the need to microbatch or round-trip data at high latencies. Use the same existing SQL to train ML models in batch - but instead adapt models in real-time.
Strict serializability
Materialize makes it simple to build a real-time feature store without sacrificing correctness. With strict serializability, you don’t need to give up correctness guarantees to train ML models with multiple data inputs.
Modern Data Applications need Modern Solutions
Machine Learning workloads have different requirements than traditional analytics, but stream processing is not the answer.
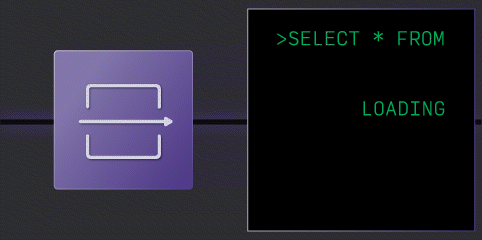
Traditional Warehouses: Too Slow
Traditional Cloud Data Warehouses are easy to use, but get expensive when run continuously, and hit hard limits on latency and concurrency.
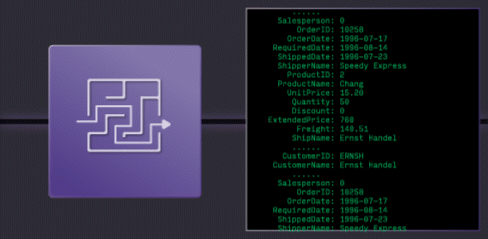
Stream Processors: Too Complicated
Stream Processors are fast, but they're a low-level tool, using them has high engineering costs, creates complex architectures.
Materialize packages the speed of stream processors in a familiar database abstraction.
Use the same SQL workflows from traditional warehouses but get results
updated continuously to power machine learning in production.
Streaming Engine
Work is done at the moment of data arrival, rather than query time, so that maintained results are available almost instantly.
PostgreSQL Serving Layer
Materialize presents as Postgres - query it with high concurrency from any tool or driver compatible with Postgres.
Access via standard SQL
Incrementally Maintained Views
Write complex SQL transformations as materialized views that efficiently update themselves as inputs change.
Learn MoreBuilt for JOINs
Multi-way, complex join support, even across databases - all in standard SQL.
Learn MoreSliding Windows
Write queries that filter to a window of time anchored to the present, Materialize will update results as time advances.
Learn MoreSQL Subscriptions
Write alerts as SQL queries with filters and subscribe to new rows as they appear.
Learn MoreCREATE MATERIALIZED VIEW my_view AS
SELECT userid, COUNT(api.id), COUNT(pageviews.id)
FROM users
JOIN pageviews on users.id = pageviews.userid
JOIN api ON users.id = api.userId
GROUP BY userid;
userID | api_calls | pageviews |
---|---|---|
VPLaKV | 400 | 20 |
MN37Mt | 60 | 9 |
1fT4KY | 72 | 42 |
sT4QY | 10 | 342 |
Incrementally Maintained Views
Write complex SQL transformations as materialized views that efficiently update themselves as inputs change.
Learn MoreCREATE MATERIALIZED VIEW my_view AS
SELECT userid, COUNT(api.id), COUNT(pageviews.id)
FROM users
JOIN pageviews on users.id = pageviews.userid
JOIN api ON users.id = api.userId
GROUP BY userid;
userID | api_calls | pageviews |
---|---|---|
VPLaKV | 400 | 20 |
MN37Mt | 60 | 9 |
1fT4KY | 72 | 42 |
sT4QY | 10 | 342 |
Built for JOINs
Multi-way, complex join support, even across databases - all in standard SQL.
Learn MoreSELECT DISTINCT ON (auctions.id)
bids.amount,
auctions.item,
auctions.seller
FROM auctions, bids
WHERE auctions.id = bids.auction_id
ORDER BY auctions.id,
bids.amount DESC,
bids.buyer;
amount | item | seller |
---|
Sliding Windows
Write queries that filter to a window of time anchored to the present, Materialize will update results as time advances.
Learn MoreCREATE MATERIALIZED VIEW my_window AS
SELECT date_trunc('minute', received_at),
COUNT(*) as order_ct, SUM(amount) as revenue
FROM orders
WHERE mz_now() < received_at + interval '5 minutes'
GROUP BY 1;
minute | order_ct | revenue |
---|
SQL Subscriptions
Write alerts as SQL queries with filters and subscribe to new rows as they appear.
Learn MoreSUBSCRIBE (
SELECT userID, email, MAX(orders.id) AS last_order
FROM users
JOIN orders ON orders.userID = users.id
GROUP BY userId, email
HAVING SUM(is_fraud) / COUNT(orders.id)::FLOAT > 0.5
);
userID | last_order | |
---|---|---|
REOtIb | a@gmail.com | 13/12/2025 |
Y5KBE8 | b@yahoo.com | 9/12/2025 |
Wj7JQ0 | c@hotmail.com | 13/12/2025 |
tPCQ0 | d@xyz.com | 13/11/2025 |
Built for JOINs
Multi-way, complex join support across real-time streams - all in standard SQL.
Active Replication
Use replication to increase availability, reduce downtime, scale seamlessly.
Secure and Compliant
SOC 2 Type 2 compliant, encrypted at rest, secure connectivity to your infra.
The Warehouse-Native Approach
to Machine Learning
You don't need to compromise on speed, data quality, control, or simplicity.
“Building an ML pipeline requires stitching multiple systems together”
Current models for machine learning operations put a ton of burden on the user - managing separate systems for raw data collection, feature storage, processing, and consumption. Materialize manages all of those pieces in a single streaming database.
“We need to train our machine learning models faster”
Data warehouses power many machine learning use cases - but can only work in batches. Materialize incrementally maintains the results of SQL queries in real-time so machine learning models never train off of old data.
“Machine learning operations requires hiring for a specialized set of skills”
Hard-coded logic requires a ton of effort to update and maintain as business requirements shift. Materialize allows you to adjust and test using standard SQL, saving time both in the short and long term.
“We can manage our feature store with our data warehouse”
Data warehouses are helpful for storing historical features as an offline feature store. With Materialize, models can be trained and served in real-time as an online feature store - and historical models can be enriched by sinking already-generated features into your data warehouse.
“We need to train our models with multiple data inputs and can’t move to streaming”
Materialize supports cross-stream and multi-way joins, without the need to microbatch or round-trip data at high latencies. Focus on what you want to build, and Materialize will handle how to get it.
“Moving to real-time training will results in errors from eventual consistency”
Don’t give up correctness guarantees for speed. All results from Materialize reflect correct answers, and models should never be falsely impacted by late-arriving labels.
More Use Cases
Real-Time & User-Facing Analytics
→Dashboards and data products need to be reactive to up-to-the-minute changes in your business.
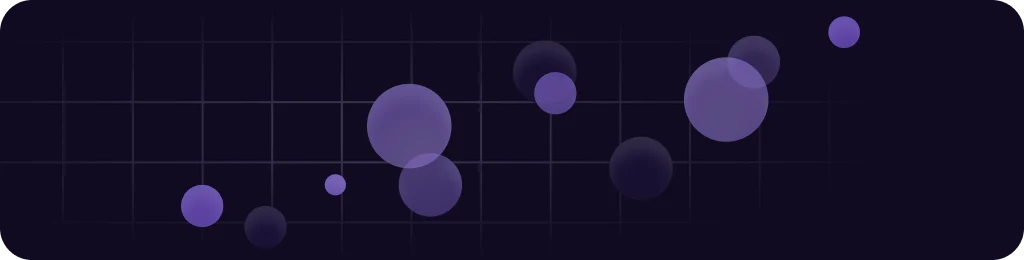
Real-time Fraud Detection
→Monitor transactions as they occur and stop fraud in seconds.
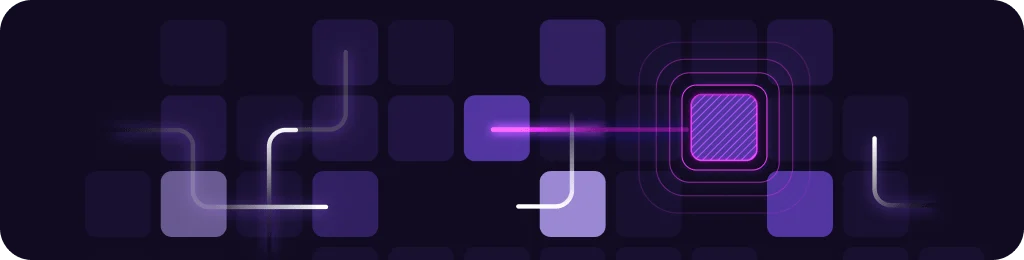
Automation and Alerting
→Save time for your users, and build value by taking action or notifying at only the right moments.
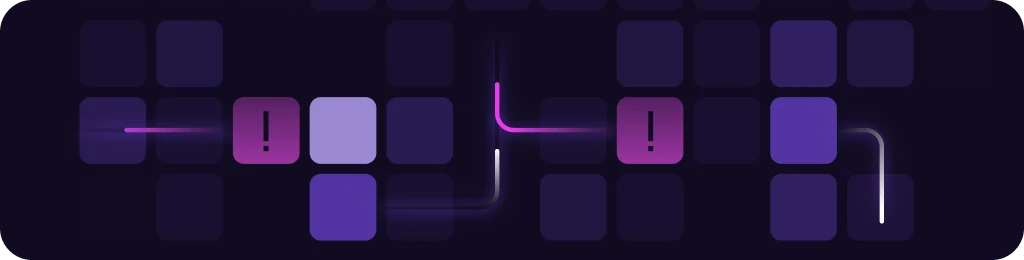
Segmentation and Personalization
→Value of personalization, recommendations, dynamic pricing increases as latency of data aggregations approaches zero.
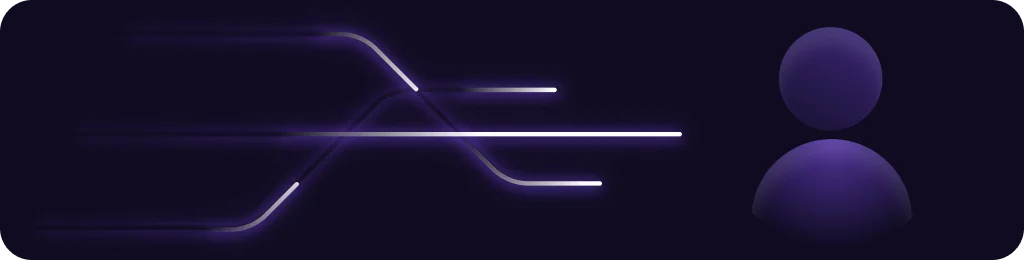