Changelog
New features and improvements landing in and around Materialize
dbt adapter: automated blue/green deployments
ICYMI: we’ve been working on supporting blue/green deployments in Materialize, which allow you to deploy schema changes to production without downtime. Now — safely cutting over to a new version of your data model in a continually running system takes a non-trivial amount of coordination…and we wouldn’t want to burden you with that.
To make blue/green deployments easier to manage, we built the workflow
into the dbt-materialize
adapter.
important
Note: The latest release of dbt-materialize
(v1.7.6), which allows
including sources in blue/green deployments, introduced a breaking change
to the syntax of source
and sink
materializations. You must migrate your
models to the new syntax before upgrading! ✋
Blue/green deployment workflow
As a recap: in a blue/green deployment, you first deploy your code changes to a
deployment environment (green
) that is a clone of your production
environment (blue
), so you can validate the results without causing
unavailability. These environments are later swapped transparently.
We baked each step of this workflow into dbt macros that you can invoke in sequence to perform a deployment:
dbt run-operation deploy_init # Create a clone of your production environment
dbt run --vars 'deploy: True' # Deploy the changes to the new deployment environment
dbt run-operation deploy_await # Wait for all objects in the deployment environment to be hydrated (i.e. lag < 1s)
# Validate the results (important!)
dbt run-operation deploy_promote # Swap environments
Behind the scenes, these macros take care of details like preserving object permissions, preventing against possibly destructive changes (e.g. cutting over too soon, concurrent operations), and letting you know when it’s safe to promote your changes. For a full rundown of the workflow, and each workflow step, check out the brand new dbt development guide in the Materialize documentation!
source
and sink
models
Breaking change: new syntax for To allow including sources in the blue/green deployment workflow, we finally
came around to making the syntax of source
(and sink
) materializations a
little uglier, but much more consistent with the other materialization types.
The new syntax omits the CREATE { SOURCE | SINK }
clause, and now accepts
configuration options like cluster
(🤝).
Please adjust your models accordingly before upgrading to the latest version of
the dbt-materialize
adapter (v1.7.6)!
New syntax
{{ config(
materialized='source',
cluster='quickstart'
) }}
FROM KAFKA CONNECTION kafka_connection (TOPIC 'test-topic')
FORMAT BYTES
Old syntax
{{ config(
materialized='source'
) }}
CREATE SOURCE {{ this }} IN CLUSTER 'quickstart'
FROM KAFKA CONNECTION kafka_connection (TOPIC 'test-topic')
FORMAT BYTES
To upgrade, run:
pip install --upgrade dbt-materialize`
And remember to migrate your source
and sink
models! If you have any
feedback on this new workflow, or requests for new features, ping our team on Slack. 🫡
New source overview UI ✨
We’ve made it easier to keep a pulse on the health of your sources right in the
web console: instead of running lengthy introspection queries by hand, you can
now monitor ingestion progress (or lack thereof) and cluster resource
utilization using the new Overview
page in the Sources
navigation tab.
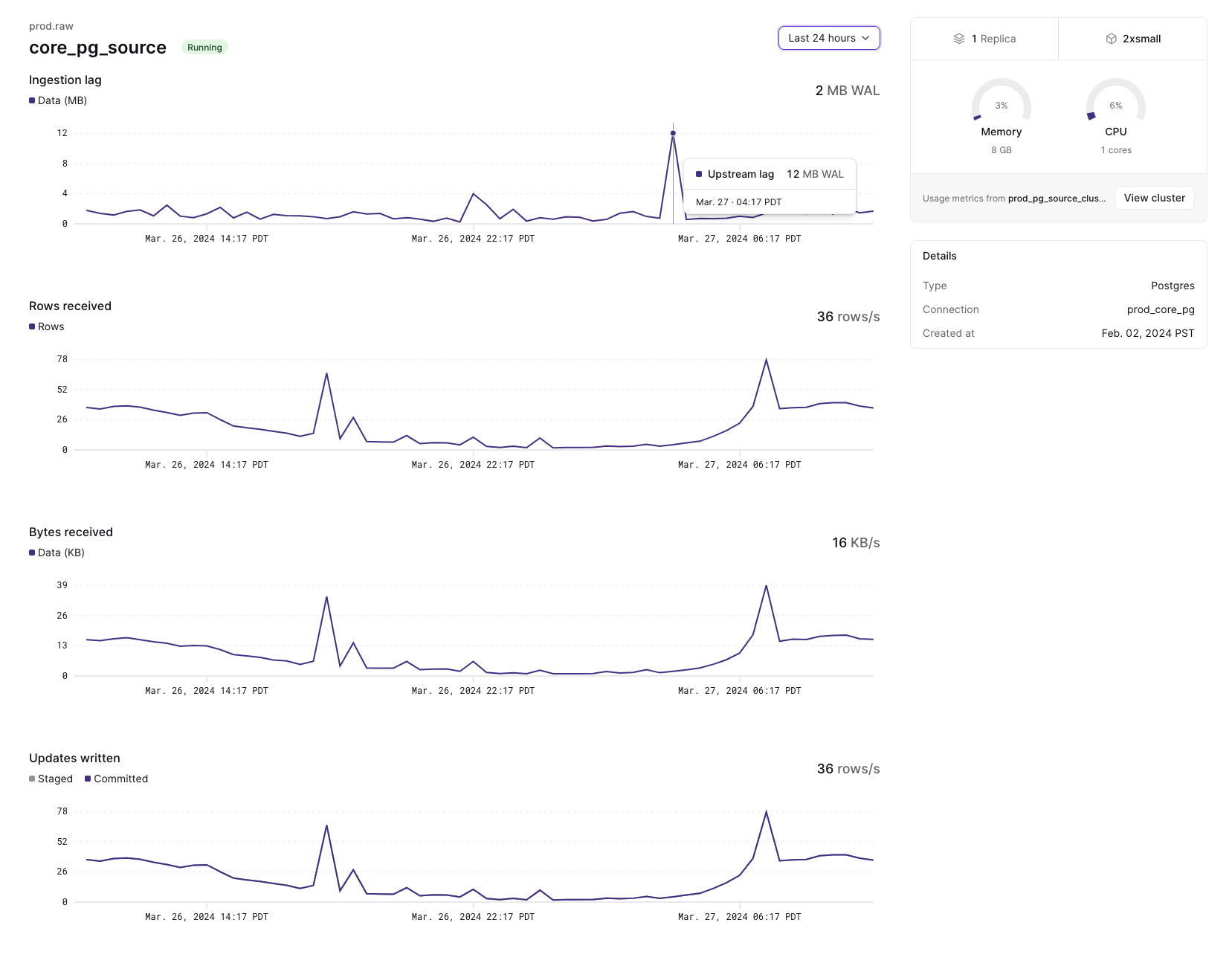
We’ll extend this overview with more details in the near future, like snapshot progress metrics, so you never miss a bit (ha!). If you have any feedback, or requests for new useful metrics, we’d love to hear about it!
Private Preview: MySQL source
Today, we’re dialing up the number of native CDC sources to two with the release of a shiny new MySQL source! 🐬 The new source allows you to replicate data from your MySQL database to Materialize in real time, and start building interactive data apps, better customer experiences, and other use cases ripe for fresh data.
How does it work?
The source uses MySQL’s binlog replication protocol to continually ingest
changes resulting from INSERT
, UPDATE
and DELETE
operations in your
upstream database. As a MySQL user, this comes with a few benefits:
No additional infrastructure: ingest MySQL change data into Materialize in real time with no architectural changes or additional operational overhead.
Transactional consistency: we’re all about correctness, so the new source ensures that transactions in MySQL are respected downstream. Materialize will never show partial results based on partially replicated transactions.
Incrementally updated materialized views: materialized views are not supported in (vanilla) MySQL, so you can use Materialize as a read-replica to build views on top of your MySQL data that are efficiently maintained and always up-to-date.
How do you get started?
Once you configure your upstream database for replication, setting up a MySQL source in Materialize really just takes three SQL statements:
CREATE SECRET mysqlpass AS '<MYSQL_PASSWORD>';
CREATE CONNECTION mysql_connection TO MYSQL (
HOST 'instance.foo000.us-west-1.rds.amazonaws.com',
PORT 3306,
USER 'materialize',
PASSWORD SECRET mysqlpass
);
CREATE SOURCE mz_source
FROM MYSQL CONNECTION mysql_connection
FOR ALL TABLES;
We aim to bring this down to zero SQL statements by supporting MySQL source creation in the Materialize console soon! For an overview of what is and isn’t supported in Private Preview, as well as integration guides for common MySQL managed services, check out the documentation.
Ready to give it a go? Sign up for a 14-day free trial of Materialize, and ping our team on Slack to get early access to the feature!
New integrations gallery 🔌
No data warehouse is an island, and Materialize is no different. To allow you to more easily browse the ecosystem of tools that integrate well with Materialize, we just shipped an integrations gallery to the web console!
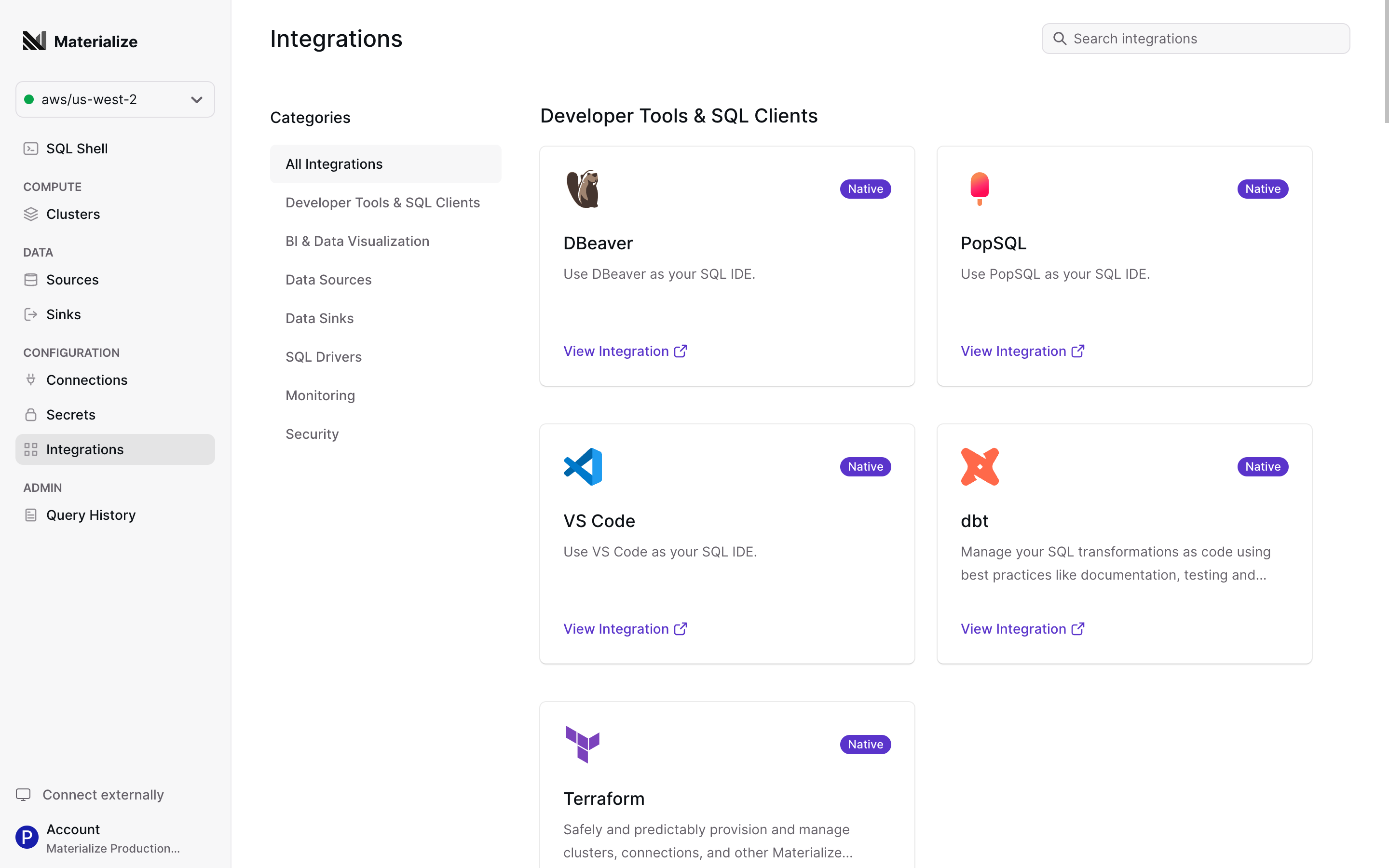
Each integration points to a documentation guide with step-by-step instructions to walk you through getting started. Not sure where to start? Here’s a quick roundup of some of the tools we support and love:
The
dbt-materialize
adapter, which allows you to manage your SQL transformations as code using best practices like documentation, testing and version control.The Materialize Terraform provider, which allows you to safely and predictably provision and manage clusters, connections, and other Materialize resources as code.
Major managed PostgreSQL services, like Amazon RDS, Google Cloud SQL or AzureDB, which allow you to ingest data in real-time using Change Data Capture (CDC).
Real-time friendly BI tools like Metabase, and interactive data notebooks like Hex or Deepnote.
And many more! From message brokers to real-time reverse ETL, we’ve got you covered. If you have any feedback, or requests for new integrations, we’d love to hear about it!
Usage & Billing
Usage & Billing is now available in the admin section of the Console! This feature provides full visibility into usage and cost trends.
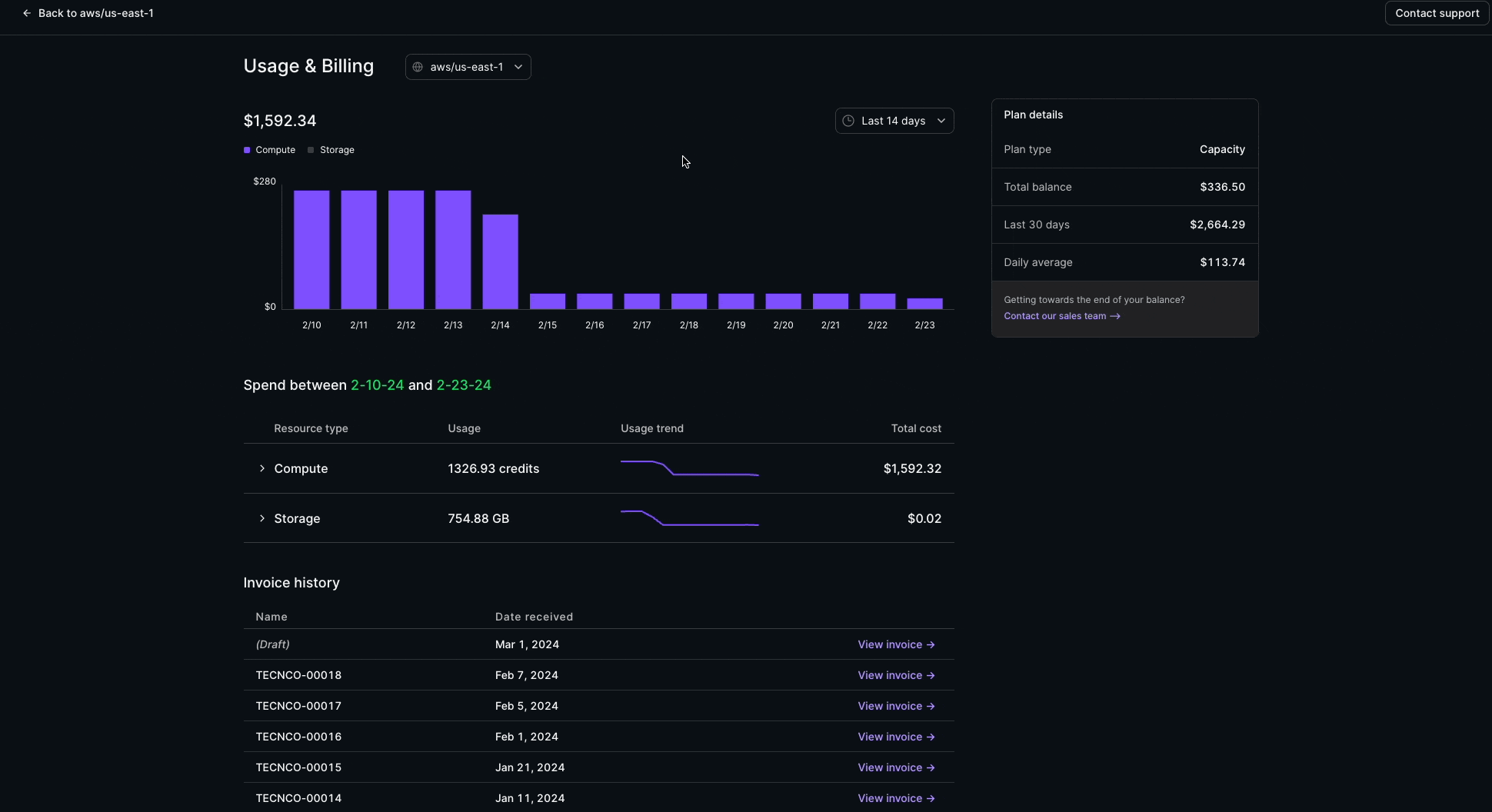
The Usage & Billing page provides a complete view into:
- Compute and Storage consumption
- Dollar and usage-based spend patterns
- Capacity balance
- Current and past monthly invoices
This feature is available for Organization Admin
roles on all paid Materialize plans.
Query History 🔍
We’ve added a modern interface to the Console for monitoring your query performance: Query History!
Now you can instantly answer questions about your SQL queries, such as:
- How long is a particular query taking?
- Which users or clusters are issuing the most expensive queries and when?
- Which applications are requesting slow running queries?
- Has my query errored or completed? Or is it still running?
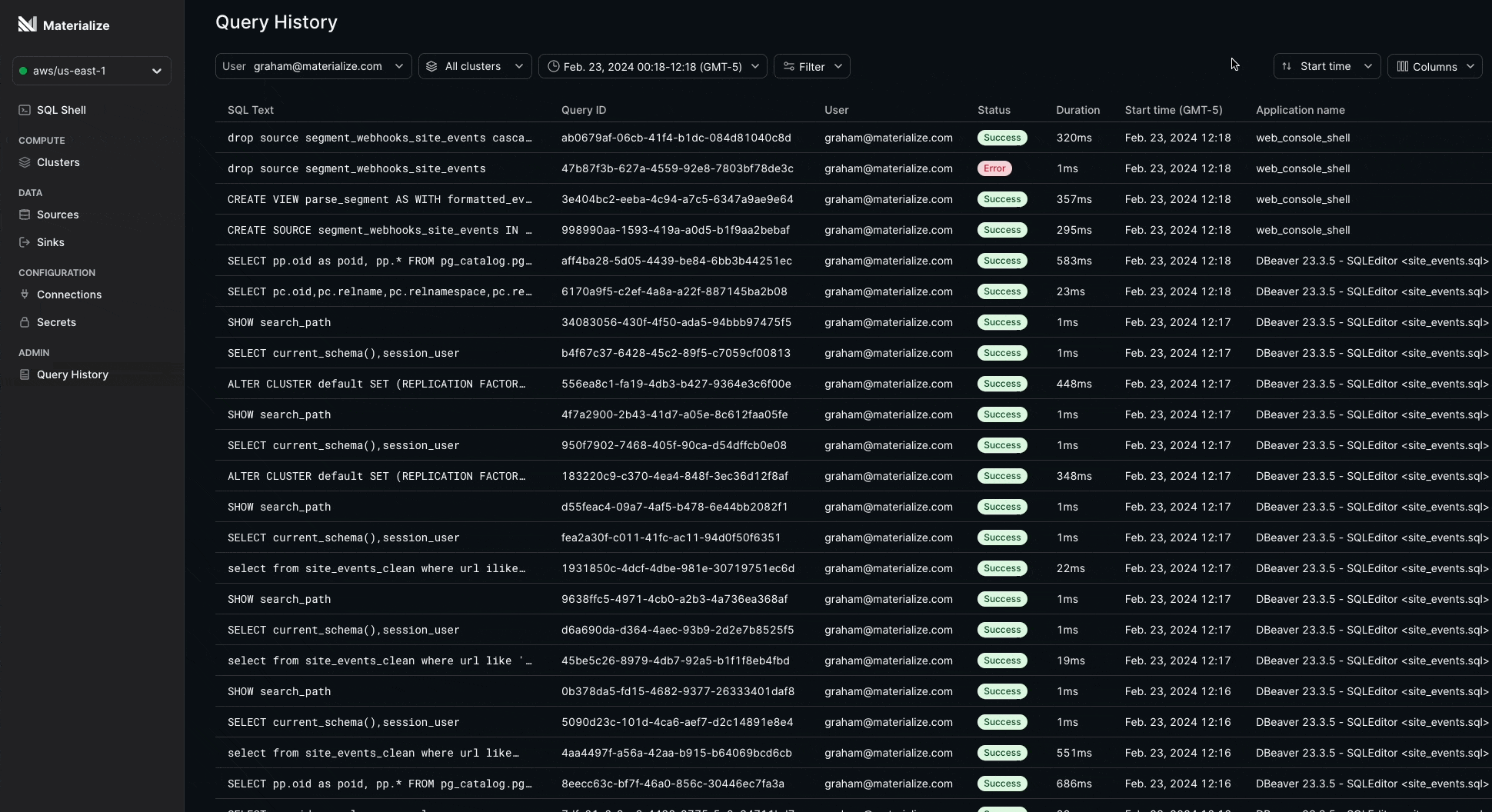
Query History is built on mz_recent_activity_log
to always deliver insights fast.
This feature is currently in Private Preview and is available for Organization Admin
and mz_monitor
roles. Contact your account team to request early access.
Webhook source: support for batched events
Many SaaS applications support webhooks as a way to push events to other applications (like Materialize) as they occur. To maximize the volume of events that can be sent at once, it’s common for these applications to batch multiple events into a single network request — and we strive to support what’s common!
The webhook source can now automatically unwrangle batched events in the following formats:
JSON arrays
If you’re expecting events batched as a JSON array, use BODY FORMAT JSON ARRAY
. Each object will be appended to the source as an individual row.
CREATE SOURCE webhook_json_array IN CLUSTER quickstart FROM WEBHOOK
BODY FORMAT JSON ARRAY;
-- Send multiple events in a JSON array to the webhook source.
HTTP POST TO 'webhook_json_array'
[
{ 'event_type': 'a' },
{ 'event_type': 'b' },
{ 'event_type': 'c' }
]
-- 🎉
SELECT COUNT(*) FROM webhook_json_array;
3
Newline-delimited JSON (aka NDJSON)
If you’re expecting events batched as NDJSON, use BODY FORMAT JSON
as you
would otherwise! Each object will be appended to the source as an individual
row.
CREATE SOURCE webhook_json IN CLUSTER quickstart FROM WEBHOOK
BODY FORMAT JSON;
-- Send multiple events delimited by newlines to the webhook source.
HTTP POST to 'webhook_json'
{ 'event_type': 'foo' }
{ 'event_type': 'bar' }
-- 🎉
SELECT COUNT(*) FROM webhook_json;
2
Besides making webhook ingestion more ergonomic, this change also allows you to take advantage of performance optimizations like temporal filter pushdown.
Configurable defaults for configuration parameters
There are details in your Materialize development workflow that you shouldn’t have to remember: the namespace or cluster you connect to by default is one of them.
To save you the hassle, it’s now possible to configure defaults for
configuration parameters
for a role using the new ALTER ROLE...SET
command. This also allows you to ensure that external tools that connect
to Materialize using non-native connectors use the right connection
parameters!
-- Say an admin configured the default cluster for **all** users to be
-- queries_brr.
SHOW cluster;
cluster
------------
queries_brr
-- As a forgetful dev, you can set your day-to-day development cluster to a
-- different default.
ALTER ROLE forgetful_dev SET cluster TO queries_dev;
-- Whenever you start a new SQL session (and only then!), you'll see that the
-- change was picked up.
SHOW cluster;
cluster
------------
queries_dev
If you’re a superuser, you can modify these defaults globally using the
ALTER SYSTEM...SET
command.
-- Important things changed, and you don't want users connecting to queries_brr
-- by default anymore? Change it for everyone at once!
ALTER SYSTEM SET cluster TO queries_skrrtt;
Cluster unification
Clusters are such an integral part of Materialize that we want to make sure you think about them as little as possible. 🧠 Over the past few months, we’ve worked on making clusters more flexible and unopinionated about what runs on them (aka cluster unification). For you, as a Materialize user, this means two things:
Depending on the characteristics of your workload, you now have the option to manage all types of objects in the same cluster. This gives you more control over capacity planning, and smooths out the experience of onboarding new users to your Materialize organization. We still recommend using separate clusters to manage ingestion and compute — but that’s a feature, not a bug now!
Although sources and sinks must be associated with a cluster, if you don’t explicitly specify one using the
IN CLUSTER
clause on creation, the objects will now be created in the active cluster for the session.--Create the object in a specific cluster CREATE SOURCE json_source IN CLUSTER some_cluster FROM KAFKA CONNECTION kafka_connection (TOPIC 'ch_anges') FORMAT JSON; --Create the object in the active cluster CREATE SOURCE json_source FROM KAFKA CONNECTION kafka_connection (TOPIC 'ch_anges') FORMAT JSON; ```
If you’re just getting started, this also means that you no longer have to learn
about cluster specifics as the Materialize 101. Off the bat, new Materialize
regions include a default cluster named quickstart
,
which does what it says on the tin: give you resources to just start building.
SCIM provisioning
You can now automatically sync users and groups from your identity provider, like Okta or Active Directory, to Materialize. Syncing is facilitated via the industry standard SCIM protocol.
To get started, visit the new Provisioning
tab in your account settings:
Capacity balance monitoring 💹
No more flying blind on your purchased capacity! You can now monitor your
current capacity balance at any time in the Materialize console, under
Invoices
. This is available to
administrators of any organization that’s on a Capacity plan.
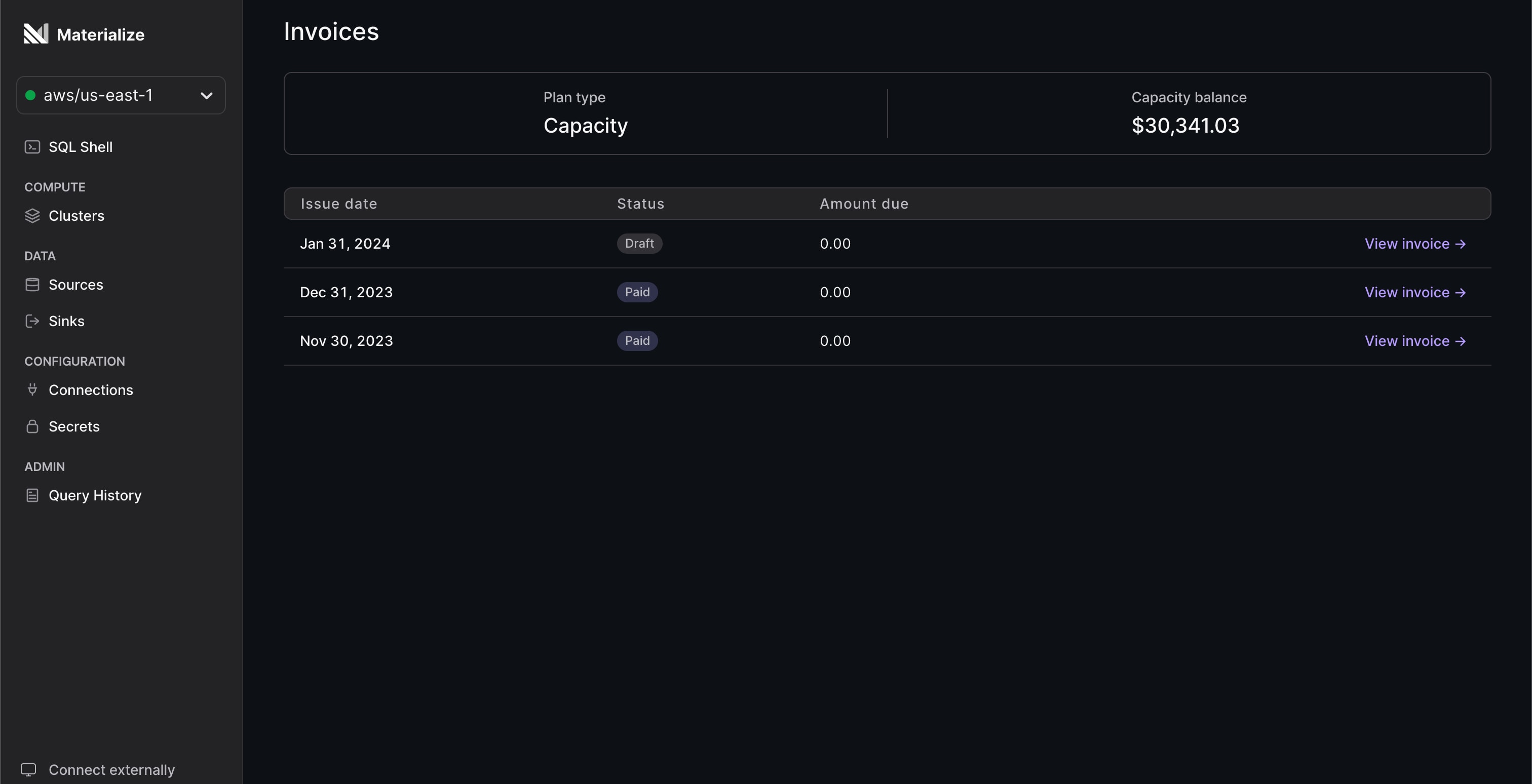
This is the first of many ongoing improvements for cost monitoring in Materialize — stay tuned for updates. If you have any feedback, or just opinions about billing observability, we’d love to hear about it!
Hello, console world!
We did some house cleaning in the Materialize console: when you log in, you’re now dropped straight into the SQL shell, so you can start interacting with your region without having to click around. You’ll notice that the connection details are now printed to the shell, too!
When you’re ready to connect using an external tool, you can find the credentials at the bottom of the navigation bar, under Connect externally.
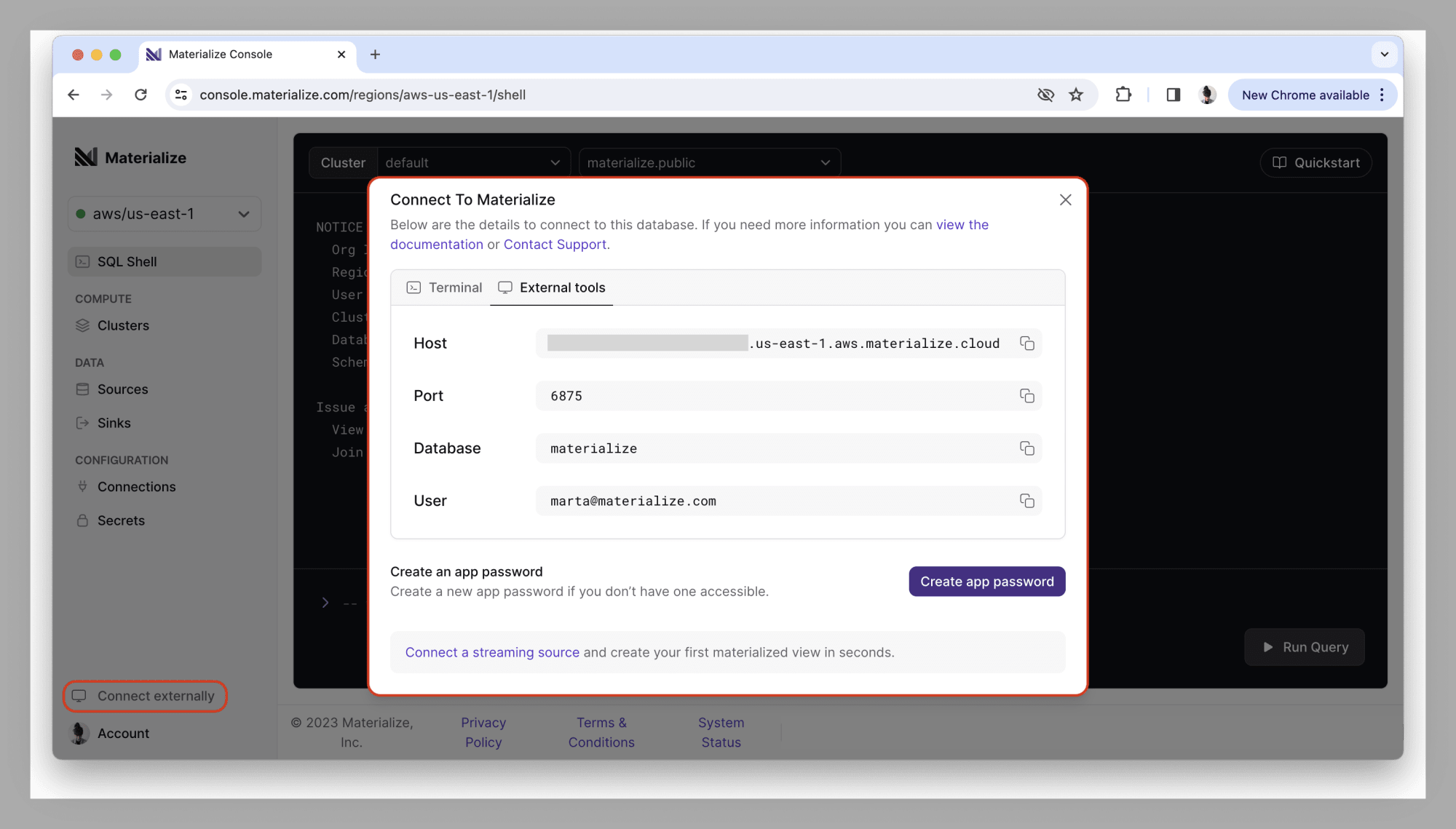
SQL activity log
Organization administrators can now access the history of SQL statements run
against Materialize using the new mz_internal.mz_recent_activity_log
system catalog view. This allows performing database administration tasks like identifying
slow or long-running queries, monitoring query performance SLAs,
and analyzing access patterns.
-- Say you have an SLA of "queries return under 3 seconds", and want to look
-- into any statements that don't comply. 🦥
SELECT
sql,
application_name,
cluster_name,
rows_returned,
(finished_at - began_at) AS execution_time,
finished_status
FROM mz_internal.mz_recent_activity_log
WHERE (finished_at - began_at) > INTERVAL '3 seconds'
ORDER BY execution_time DESC;
In the future, the activity log will also be exposed in the Materialize console, so it’s easier to access.
New Workflow graph 👀
The days of fiddling with the system catalog to understand why your queries are laggy or unresponsive are over! With the new Workflow graph freshly available in the Materialize console, you can get a visual overview of the (resource-consuming) dependencies for an object, as well as track the freshness of its data.
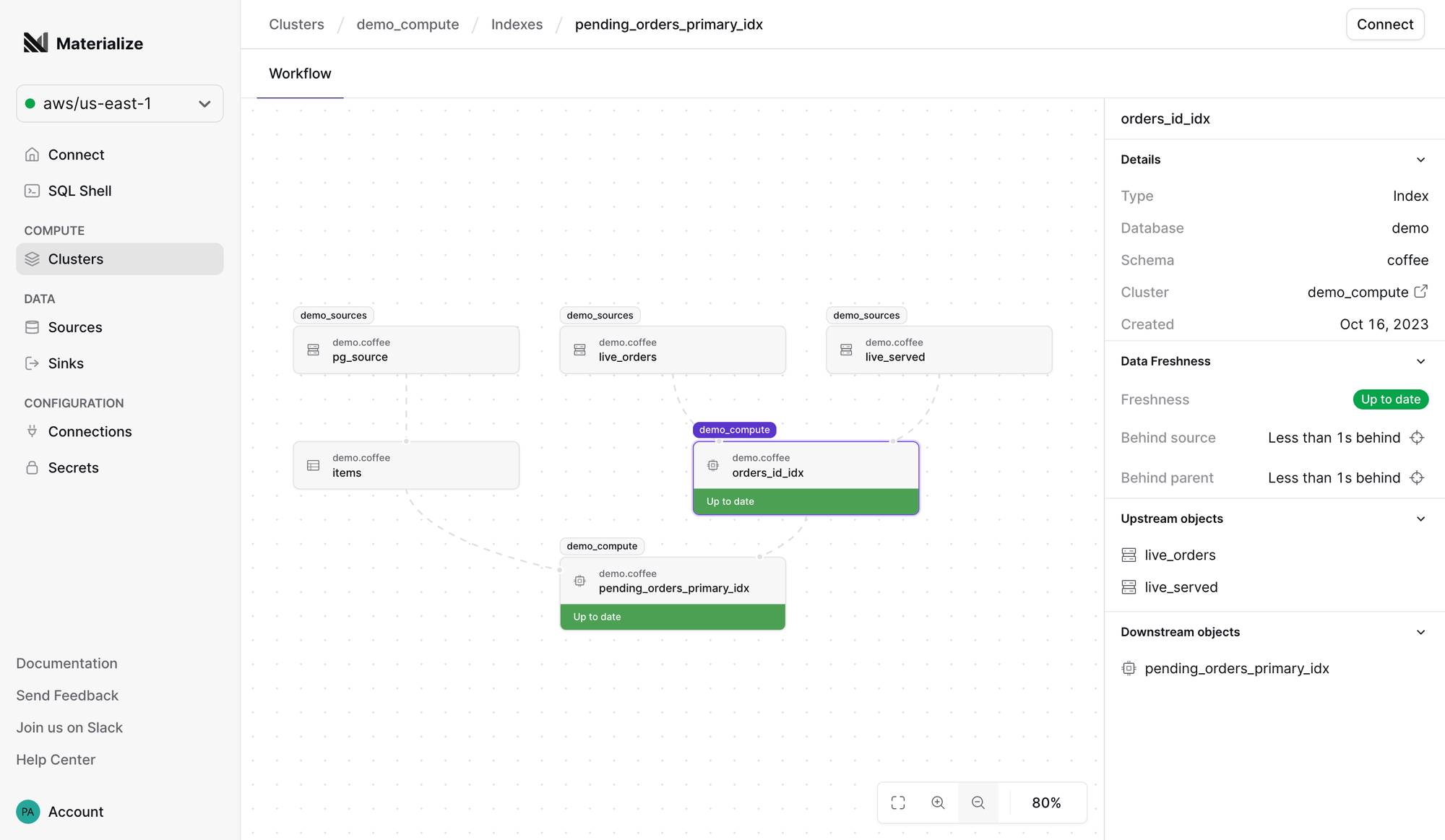
Experiencing unexpected query latency? Not getting results back at all? Open up
the Workflow
tab for the offending object, and the graph will help you
pinpoint where the bottleneck is being introduced.
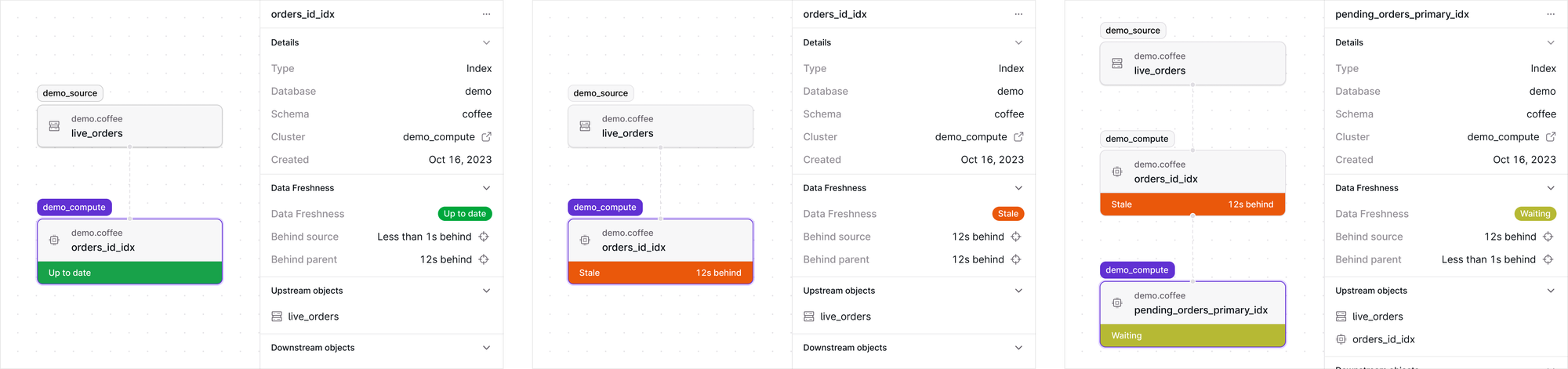
Before you start: this feature applies to all object types that require Materialize to do some work (i.e. sources, indexes and materialized views), but won’t give you an overview of the whole data model — at least, not yet! If you’re looking for a full-blown lineage graph, we recommend checking out dbt and its documentation features for the time being.
Materialize CLI
We are releasing a command-line interface (CLI) for Materialize into the wild!
The Materialize CLI (mz
) is built to improve your day-to-day workflow
for common environment management tasks, and gives you a programatic way to
manage resources like regions, users, or secrets.
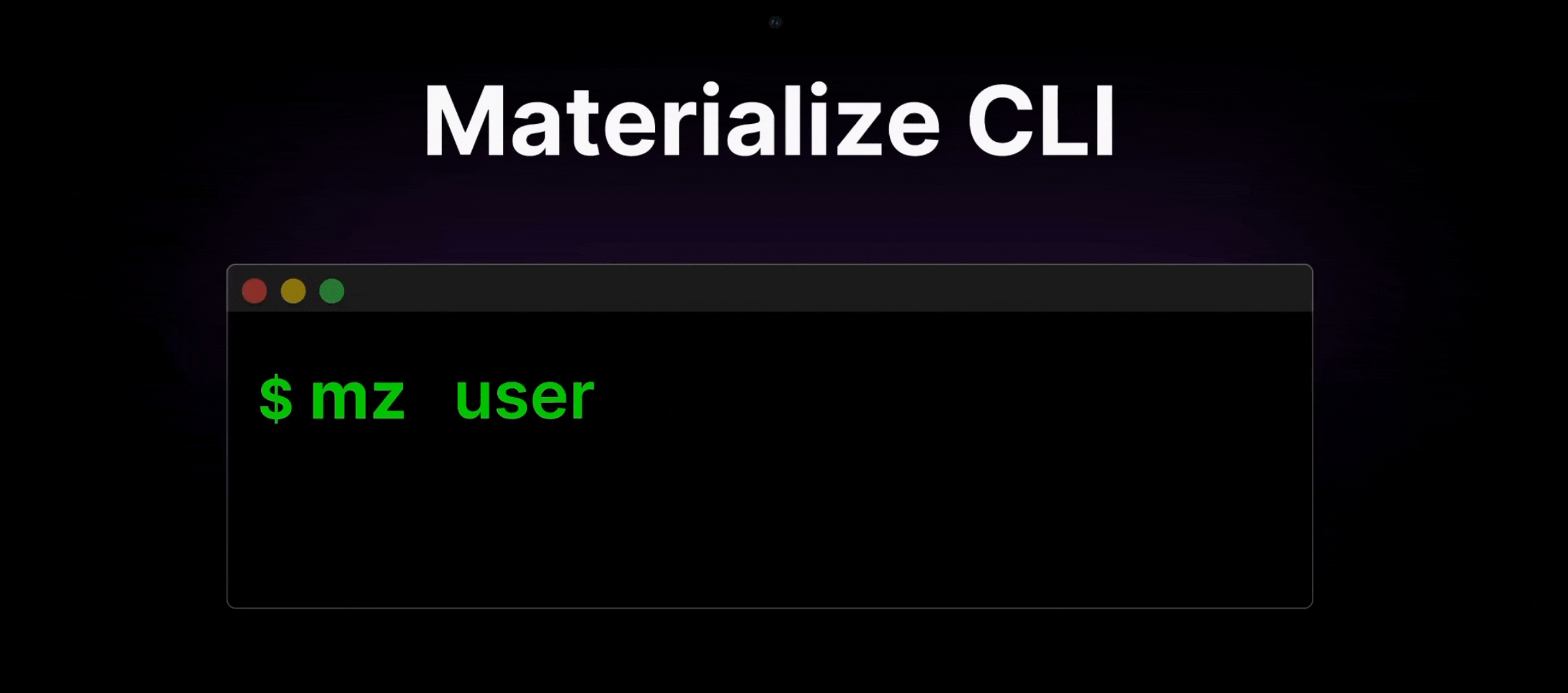
Feature highlights:
- Profiles: manage and switch between multiple authentication profiles.
- App passwords: create new, or list existing, app passwords.
- Regions: enable, disable, list, or show your region’s connection details.
- Users: add, remove, or list users in your organization.
- Secrets: create secrets without leaving a trace in your logs.
- SQL: quickly open a SQL session against your region.
Check the documentation for
installation steps and all available commands. If you have any
feedback, or just opinions about what mz
should do but doesn’t, we’d love
to hear about it!
Aggregate window functions
If you’re used to traditional data warehouses, reaching for functions like AVG ()
or SUM()
to get a rolling aggregation is second nature. Things aren’t as
simple for real-time data — as it turns out, calculating window aggregations
over ever-changing data is hard. 😰
But we’ve made it! Support for aggregate window functions is here, so you can
more naturally express common query patterns that require using an aggregate
function with an OVER
clause:
-- Hey Materialize: give me the cumulative $um of sales over time.
SELECT time,
amount,
SUM(amount) OVER (ORDER BY time) AS cumulative_amount
FROM sales
ORDER BY time;
time | amount | cumulative_amount
------+--------+-------------------
1 | 3 | 3
2 | 6 | 9
3 | 1 | 10
4 | 5 | 15
5 | 5 | 20
6 | 6 | 26
-- Need more control over the granularity of the rolling aggregation? The ROWS
-- BETWEEN clause is also supported!
SELECT time,
amount,
SUM(amount) OVER (ORDER BY time ROWS BETWEEN 2 PRECEDING AND CURRENT ROW) AS cumulative_amount
FROM sales
ORDER BY time;
time | amount | cumulative_amount
------+--------+-------------------
1 | 3 | 3
2 | 6 | 9
3 | 1 | 10
4 | 5 | 12
5 | 5 | 11
6 | 6 | 16
Head over to the documentation for an overview of window function support in Materialize.
dbt adapter: model contracts, new configuration for tests, and more!
dbt is the most popular tool to manage Materialize deployments in production
(along with Terraform), and
we do our best to keep our adapter in sync with any new features landing in
dbt-core
! With dbt-materialize
v1.6.0 freshly released, here are a few
🔥 improvements that can elevate your data deployments.
Model contracts
You can now enforce model contracts
for view
, materializedview
and table
materializations to guarantee that
there are no surprise breakages to your pipelines when the shape of the data
changes.
# Enforce a contract by using the new `contract` config. This requires that all
# columns in the model are listed with the respective name and data_type.
models:
- name: on_time_bids
description: On time auction bids
config:
contract:
enforced: true
columns:
- name: auction_id
description: ID of the auction
data_type: bigint
...
- name: amount
description: Bid amount
data_type: int
...
# If there is a mismatch between the defined contract and the model you’re trying
# to compile, dbt will fail during compilation!
16:37:58
16:37:58 | column_name | definition_type | contract_type | mismatch_reason |
16:37:58 | ----------- | --------------- | ------------- | ------------------- |
16:37:58 | bid_id | LONGINTEGER | | missing in contract |
16:37:58 | amount | TEXT | INTEGER | data type mismatch |
As a side note: Materialize does not have a notion of constraints, so model- and column-level constraints are not supported.
Cluster configuration for tests
A small but mighty change: you can now configure a target cluster to run your tests against (for both one-shot and continuous testing). This means that it’s possible to configure your models to run against a specific cluster (i.e. dedicated compute resources), and ensure that tests don’t impact the performance of your data warehouse by configuring them to use a different cluster.
tests:
example:
+store_failures: true # this is optional (and called "continuous testing")
+schema: 'dbt_test_schema'
+cluster: 'dbt_test_cluster'
New materialization name, same materialized views
The latest release of dbt-core
has introduced support for materialized
views for multiple data warehouses via the new materialized_view
materialization. Now, if you’ve been following Materialize, you’ll know that
we’ve always supported incrementally updated materialized views via the custom
materializedview
materialization. Our materialized views are still special,
and nothing really changes; we’re just adjusting the name for consistency.
New
{{ config( materialized = 'materialized_view' )}}
Deprecated
{{ config( materialized = 'materializedview' )}}
The deprecated materialization name will be removed in a future release of the adapter, so we encourage you to tweak any relevant models with this upgrade!
Query cancellation
Last but not least, we patched a long-running pet peeve that prevented query cancellation in the adapter. All you have to do now is press Ctrl+C, and any outstanding queries will be ☠️.
To upgrade to the latest version of the dbt-materialize
adapter (v1.6.0), run
pip install --upgrade dbt-materialize
, and take a peek at the full changelog.
If it’s your first time hearing about dbt + Materialize for real-time data
wrangling, head over to our documentation
for an intro.
Connection validation
Few things are more common than fat-fingering connection parameters. To avoid the frustrating loop of creating, dropping, and recreating your connections, Materialize now supports connection validation.
Two ways!
For most connection types, Materialize automatically runs the validation check on connection creation, so you get an error as soon as you run the DDL statement:
-- Say you mess up your PostgreSQL credentials and try to create a
-- connection
CREATE SECRET pg_password AS 'wr0nGpa$$w0rd';
CREATE CONNECTION pg_connection TO POSTGRES (
HOST 'instance.foo000.us-west-1.rds.amazonaws.com',
PORT 5432,
USER 'postgres',
PASSWORD SECRET pg_password,
SSL MODE 'require',
DATABASE 'postgres'
);
-- Not on our watch!
Error: db error: FATAL: password authentication failed for user "materialize": FATAL: password authentication failed for user "postgres"
For AWS PrivateLink
and SSH tunnel
connections, which require a more intricate set of configuration steps across
multiple systems, Materialize can’t perform this validation off the bat, but
allows you to manually validate the connection with the new
VALIDATE CONNECTION
syntax:
-- Once you're done configuring the AWS PrivateLink service and create a
-- connection
CREATE CONNECTION privatelink_svc TO AWS PRIVATELINK (
SERVICE NAME 'com.amazonaws.vpce.us-east-1.vpce-svc-0e123abc123198abc',
AVAILABILITY ZONES ('use1-az1', 'use1-az4')
);
-- Check if the setup is 👍, before using the connection to create
-- a source or sink
VALIDATE CONNECTION privatelink_svc;
If no rows are returned, you’re good to go! Configuration issues will lead to a validation error with details on where things went haywire.
Role-based access control (RBAC) 🔒
We’ve rolled out role-based access control (RBAC) so you (well, an admin) can define a hierarchy of roles and permissions for your organization. If you’re familiar with how RBAC works in PostgreSQL, Materialize largely follows the same principles — check the documentation for a refresher!
Say you want to onboard a Data Science team to your Materialize organization, but this team should only have access to a specific namespace and dedicated compute resources:
-- Create a role through which Data Science team members can inherit a specific
-- set of privileges
CREATE ROLE data_scientist;
-- Grant the Data Science team members the data_scientist role
GRANT data_scientist TO "gerald@dharma.com", "karen@dharma.com";
-- Create a dedicated namespace and spin up some compute resources for Data
-- Science team work
CREATE DATABASE actual_science;
CREATE CLUSTER for_science (SIZE = '3xsmall');
-- Grant the data_scientist role free reign over the actual_science database and
-- the for_science cluster
GRANT ALL PRIVILEGES ON DATABASE actual_science TO data_scientist;
GRANT ALL PRIVILEGES ON CLUSTER for_science TO data_scientist;
-- If a Data Science team member ever tries to do science elsewhere...
-- (e.g. running as gerald@dharma.com)
SELECT AVG(speed) FROM not_science.public.asteroids;
ERROR: permission denied for TABLE "not_science.public.asteroids"
Pretty standard, huh?
RBAC is also supported in the Materialize Terraform provider (v0.0.9+). If you’re using Terraform to manage region-level resources like connections, sources, sinks and others, you can now also manage roles, object ownership and permissions in an automated, source-controlled way.
PostgreSQL source: support adding and dropping tables
We get it: schemas change.
We’ve landed some quality-of-life improvements that make it easier to
handle schema evolution and replication errors in PostgreSQL sources.
By easier, we mean that you no longer have to drop and recreate the whole
source, but can instead use the new ALTER SOURCE...{ADD|DROP} TABLE
syntax to
patch a specific table (or set of tables).
As an example, if you add a important_col
column to the important_tbl
table
in your upstream PostgreSQL database, and want to make sure this new column
starts being ingested in the pg_source
PostgreSQL source in Materialize:
-- List all subsources in pg_source
SHOW SUBSOURCES ON pg_source;
-- Get rid of the outdated subsource
ALTER SOURCE pg_source DROP TABLE important_tbl;
-- Start ingesting the table with the updated schema
ALTER SOURCE pg_source ADD TABLE important_tbl;
The same approach works for incompatible schema changes that might cause one or more subsources to stall. For more details on how to handle each scenario, check the updated documentation.
DBeaver native driver
DBeaver users have long been able to connect to Materialize via the native
PostgreSQL connector, due to our pgwire
compatibility.
This just works™️, but can cause some confusion, as well as annoying errors that
pop up when you accidentally click a widget for features that don’t carry over
to Materialize (like triggers).
From DBeaver 23.1.3, you can connect to your Materialize region using the new native database driver:
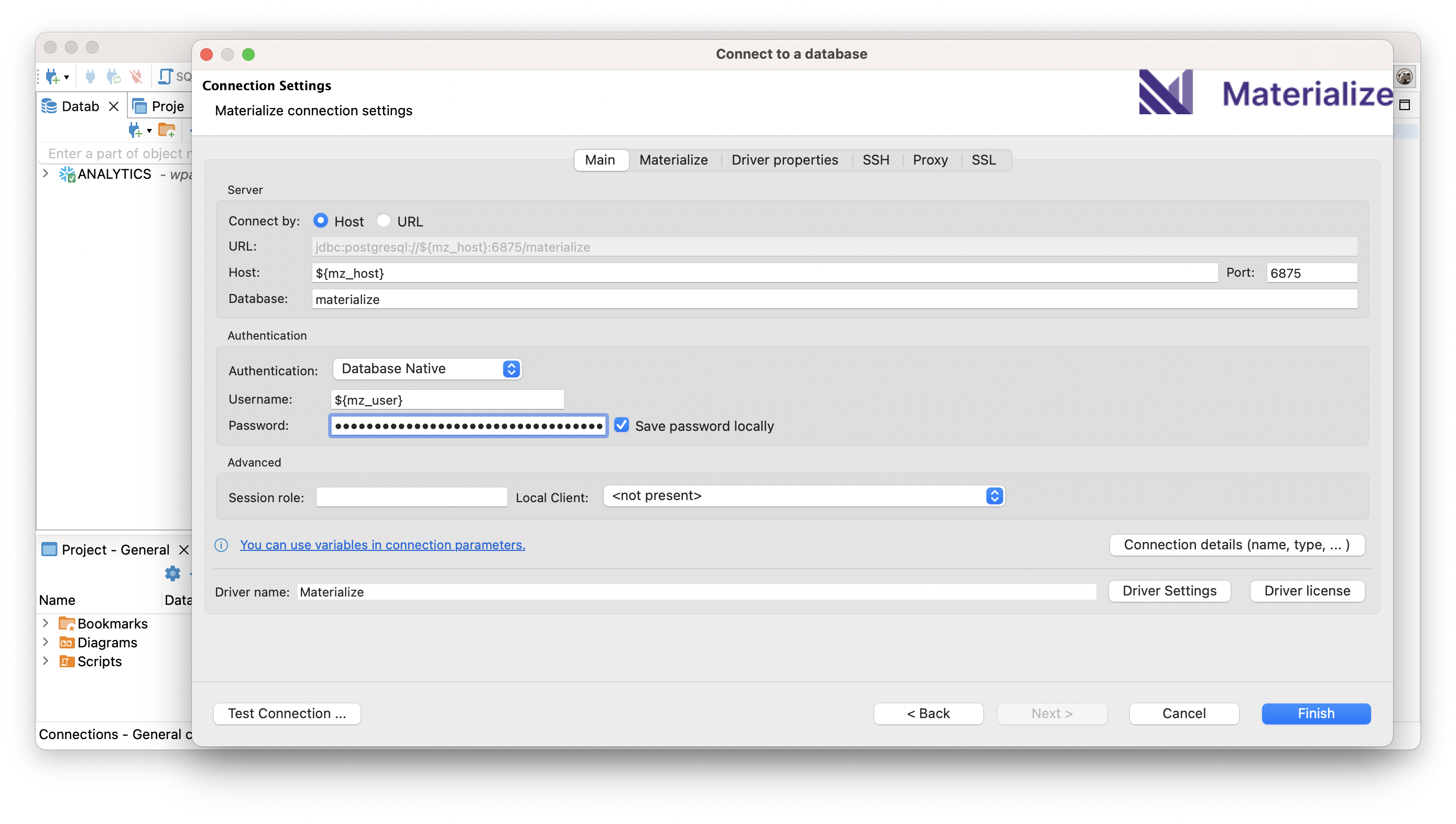
If you’re already using Materialize with DBeaver, switch over for a smoother experience! 🦫 Check the updated documentation to connect.
New SQL shell 🐚
We’re lifting the veil on a shiny, new SQL shell that lets you interact with Materialize right in the console! Neat, huh?
Although this isn’t a replacement for your everyday SQL development environment (at least for now), it’s just…right there. No more context switching just to learn your way around Materialize, run sanity checks, or pull up that data real quick. If you have any feedback, or just opinions about what the SQL shell should do but doesn’t, we’d love to hear about it!
Want to give the new SQL shell a go? Sign up for a 14-day free trial of Materialize.
Kafka source: improved JSON support
Handling JSON-encoded Kafka topics in Materialize isn’t fun. We’ve taken the
first step to improve JSON support in the Kafka source by introducing a new
FORMAT JSON
option
that decodes messages as jsonb
, saving you some conversion typing in
comparison to FORMAT BYTES
.
The old way
CREATE SOURCE json_source
FROM KAFKA CONNECTION kafka_connection (TOPIC 'ch_anges')
FORMAT BYTES
WITH (SIZE = '3xsmall');
CREATE VIEW extract_json_source AS
SELECT
(data->>'field1')::boolean AS field_1,
(data->>'field2')::int AS field_2,
(data->>'field3')::float AS field_3
-- This is not fun
FROM (SELECT CONVERT_FROM(data, 'utf8')::jsonb AS data FROM json_source);
The new way
CREATE SOURCE json_source
FROM KAFKA CONNECTION kafka_connection (TOPIC 'ch_anges')
FORMAT JSON
WITH (SIZE = '3xsmall');
CREATE VIEW extract_json_source AS
SELECT
(data->>'field1')::boolean AS field_1,
(data->>'field2')::int AS field_2,
(data->>'field3')::float AS field_3
-- This is slightly more fun!
FROM json_source;
The FORMAT JSON
option is already supported in the source creation UI,
and will land in the upcoming v0.1.0
release of the Terraform provider.
In the future, we plan to support automatically enforcing JSON schemas, both for
user-provided schemas and schemas managed via Confluent Schema Registry.
Cluster management (revisited)
Clusters are one of the very first concepts you run into when getting started with Materialize — you need compute resources to run your queries, right? We’ve simplified how clusters and their dedicated resources (aka replicas) are exposed, so they’re more intuitive to manage.
To create a new cluster, you can now simply specify its SIZE
and REPLICATION FACTOR
, instead of thinking about individual replicas for provisioning and
replication. Here’s a quick tour of how cluster management works from hereon:
-- Create a cluster with provisioned resources
CREATE CLUSTER demo SIZE = '3xsmall';
--Resize the cluster
ALTER CLUSTER demo SET (SIZE = 'small');
--Increase the replication factor for fault tolerance
ALTER CLUSTER demo SET (REPLICATION FACTOR=2);
-- Turn off the cluster for the night, to save $$!
ALTER CLUSTER demo SET (REPLICATION FACTOR=0);
You can still choose to manage the replicas in a cluster manually, for example to gracefully resize it without downtime, but the bottom line is: you shouldn’t have to! We’ll continue working on improving cluster management, and are eyeing features like zero-downtime resizing, autoscaling, and automatic rebalancing across availability zones for future releases. Head over to the documentation for the full rundown!
New source creation UI ✨
We’ve made it easier to connect to external data sources right in the web console: with just a few clicks, you can configure a connection and start ingesting data into Materialize using the new source creation UI. Look ma, no DDL:
For now, this workflow is restricted to PostgreSQL and Kafka sources that don’t require network security configuration. We’ll add support for configuring PrivateLink and SSH connections via the web console next! Until then, you can fire up a SQL client and head over to our documentation for step-by-step network security guides.
The Materialize Changelog is here!
A lot has changed since we rolled out the next generation of Materialize (v0.27). But how would you know? We’re starting a changelog to keep you up to speed with all new features and improvements landing in and around Materialize!