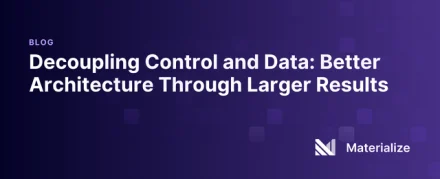
Jul 3, 2025
Decoupling Control and Data: Better Architecture Through Larger Results
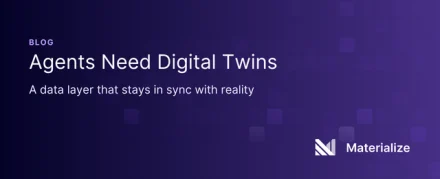
Jun 30, 2025
Agents Need Digital Twins
AI agents need a scalable data layer that stays in sync with reality. Create real-time digital twins and expose them through model context protocol (MCP) to make your data agent-ready.
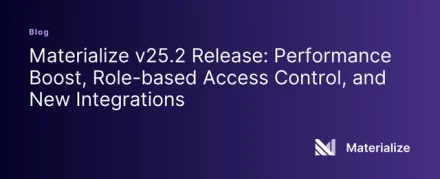
Jun 25, 2025
Introducing Materialize v25.2: Enhanced Performance, Security, and Observability
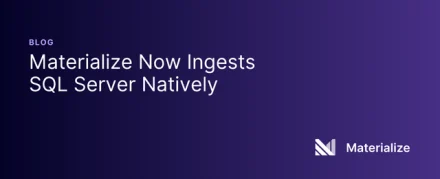
Jun 2, 2025
Materialize Now Ingests SQL Server Natively
And for the first time, you can build always-correct, always-current operational data products on top of SQL Server.
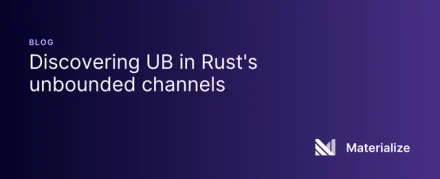
May 15, 2025
Diagnosing a Double-Free Concurrency Bug in Rust's Unbounded Channels
Explore how Materialize engineers diagnosed and resolved a rare concurrency bug in Rust's unbounded channels that led to undefined behavior through double-free memory errors.
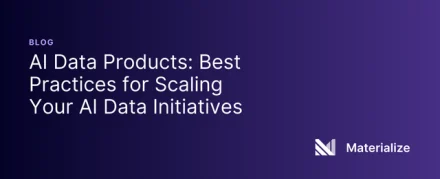
May 8, 2025
AI Data Products: Best Practices for Scaling Your AI Data Initiatives
Data products provide context and relations for data assets. Learn how tools like Materialize can help you deploy them for AI development.
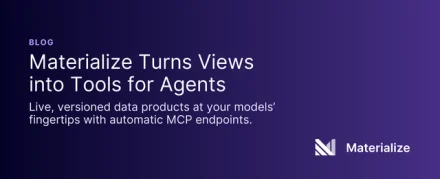
May 7, 2025
Materialize Turns Views into Tools for Agents
Live, versioned data products at your models' fingertips with automatic MCP endpoints.
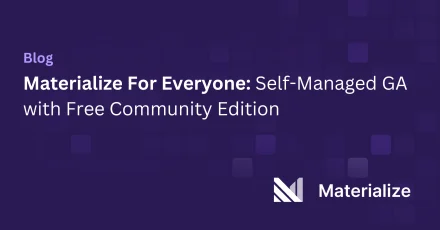
Mar 11, 2025
Materialize For Everyone: Introducing Self-Managed and our Free Community Edition
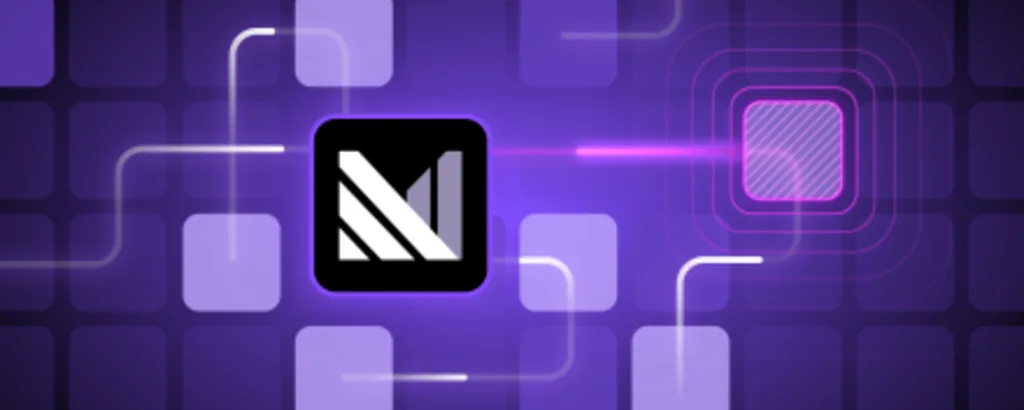
Feb 21, 2025
Why AI Systems Fail—And How Real-Time Data Fixes Them
Comprehensive privacy policy details for Materialize users, focusing on data use & protection.
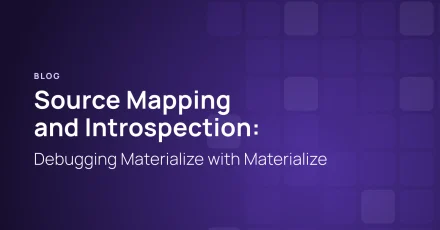
Jan 30, 2025
Source Mapping and Introspection: Debugging Materialize with Materialize
Materialize now exposes source maps in its catalog, so you can build your own debugging queries that attribute performance characteristics to high-level operators.
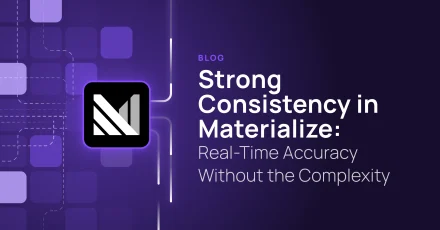
Jan 24, 2025
Materialize's Strong Consistency Guarantees for Continually Changing Data
Learn how Materialize brings order to views over independent, continually changing, transactional data sources
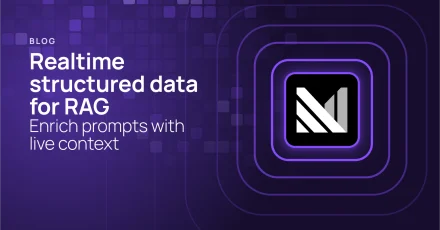
Jan 16, 2025
Real-Time Structured Data for RAG: Enrich prompts with live context
Discover how real-time structured data elevates Retrieval-Augmented Generation (RAG) systems. Learn how Materialize ensures fresh, consistent views of operational data to power context-aware, actionable AI.
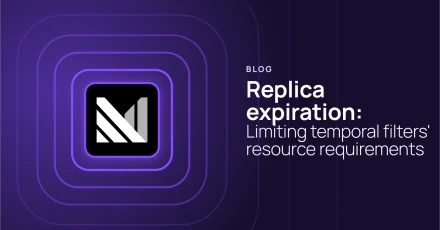
Jan 15, 2025
Replica expiration: Limiting temporal filters' resource requirements
Replica expiration is a new feature in Materialize that limits the resource requirements of temporal filters.
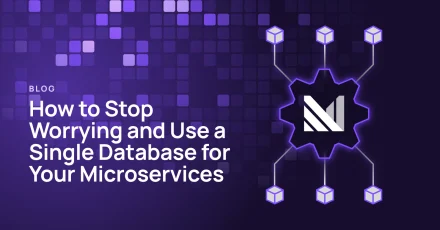
Jan 14, 2025
How to Simplify Microservices with a Shared Database and Materialized Views
Learn how to simplify microservices data integration by using a shared database without sacrificing performance or scalability. Discover how materialized views can enable consistent, efficient data access across services.
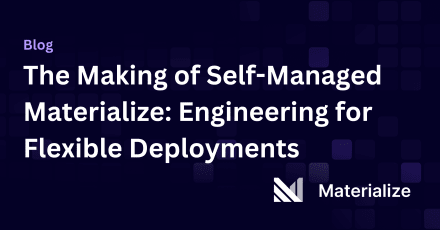
Dec 17, 2024
The Making of Materialize Self-Managed: Flexible Deployments Explained
Discover how Materialize engineered its self-managed product to support flexible deployments, improve architecture, and meet diverse customer needs, all while refining its managed cloud service.
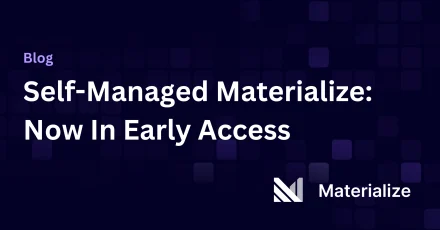
Dec 16, 2024
Materialize Self-Managed: Early Access Now Available
Get early access to Materialize Self-Managed and run it within your private infrastructure. Meet governance, compliance needs, and deploy in any cloud with Materialize's real-time data transformation capabilities.
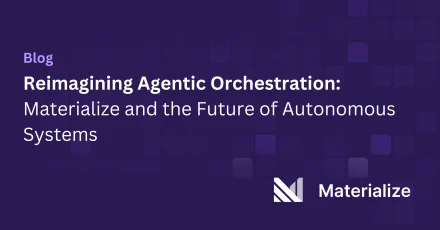
Dec 13, 2024
Reimagining Agentic Orchestration: Materialize and the Future of Autonomous Systems
Discover how Materialize empowers intelligent agents to collaborate in real-time, ensuring cost-effective and efficient orchestration for autonomous systems. Transform the future of AI-powered ecosystems with fresh, consistent, and actionable insights.
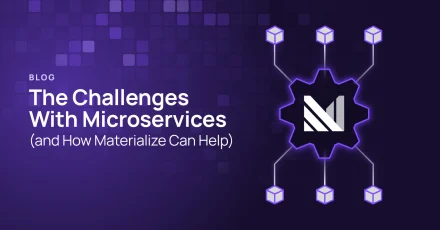
Dec 11, 2024
The Challenges With Microservices (and how Materialize can help)
Explore how Materialize overcomes key microservices challenges like data silos, network fan-out, and reconvergence issues. Learn how database-level transformations unlock real-time, consistent, and efficient operations in microservices architectures.
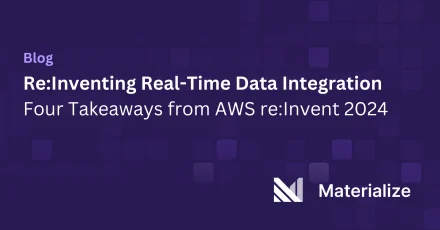
Dec 9, 2024
Re:Inventing Real-Time Data Integration
Four Takeaways from AWS re:Invent 2024
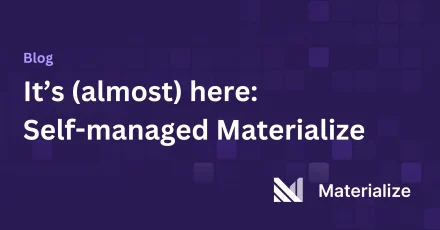
Nov 25, 2024
It’s (almost) here: Materialize Self-Managed
A new way to run Materialize in the cloud for organizations with unique operational requirements. Join the Early Access program today!
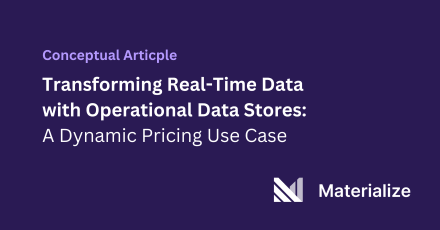
Oct 23, 2024
Transforming Real-Time Data with Operational Data Stores: A Dynamic Pricing Use Case
To showcase the power of an ODS, we’ve developed a demo for an e-commerce company, based on a dynamic pricing use case.
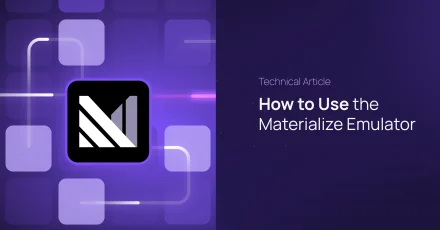
Oct 10, 2024
How to Use the Materialize Emulator
Here's a step-by-step walkthrough of how to use the Materialize Emulator.
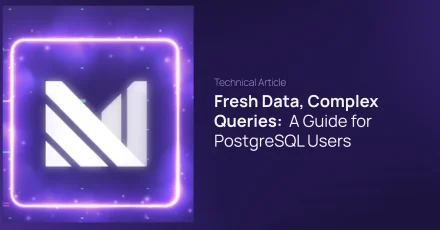
Oct 4, 2024
Fresh Data, Complex Queries: A Guide for PostgreSQL Users
Let's explore why many teams rely on PostgreSQL for analytics, the challenges they face, and how Materialize solves these problems.
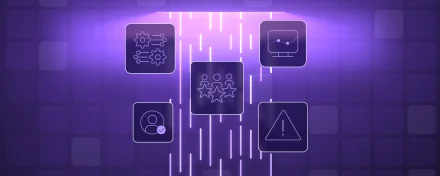
Oct 2, 2024
Migrating from dbt-postgres to dbt-materialize
In this guide, we’ll show you how to migrate your existing PostgreSQL dbt project to Materialize with minimal SQL tweaks.
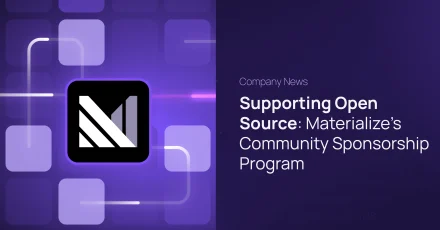
Sep 25, 2024
Supporting Open Source: Materialize’s Community Sponsorship Program
Read about how we give back to the open source community through our Community Sponsorship Program.
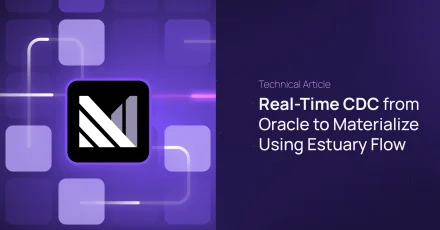
Sep 24, 2024
Real-Time CDC from Oracle to Materialize Using Estuary Flow
In this tutorial, we’ll connect Oracle CDC to Materialize in just a few minutes using Estuary Flow’s Dekaf.
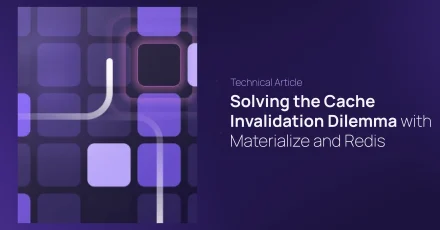
Sep 17, 2024
Solving the Cache Invalidation Dilemma with Materialize and Redis
In this post, we’ll explore the difficulties of cache invalidation, how Materialize and Redis address them, and when this solution is most effective.
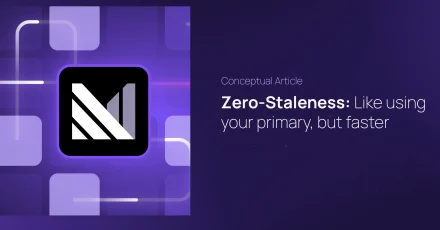
Sep 13, 2024
Zero-Staleness: Like using your primary, but faster
Materialize can respond faster than your primary database, with results that are at least as fresh as your primary would provide.
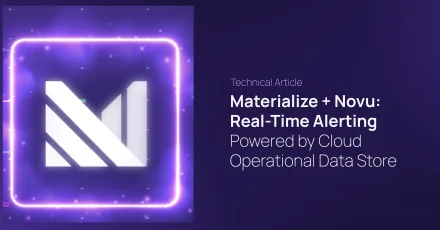
Sep 12, 2024
Materialize + Novu: Real-Time Alerting Powered by a Cloud Operational Data Store
In the following blog, we’ll show you how to create real-time alerts using Materialize’s integration with Novu.
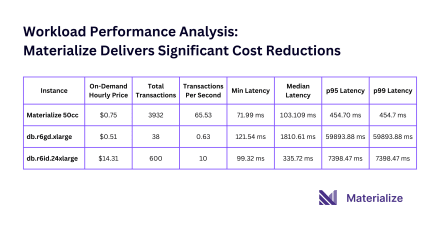
Sep 9, 2024
Materialize: More Cost-Effective than Aurora Read Replicas
Materialize costs 1/20th what Aurora PostgreSQL read replicas cost, when you have non-trivial business logic.
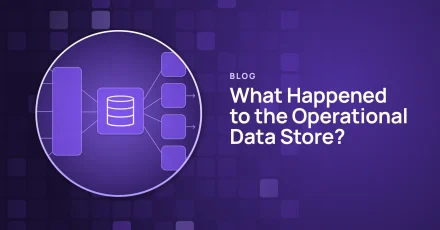
Aug 21, 2024
What Happened to the Operational Data Store?
Operational data stores maintained real-time data, and allowed access to denormalized data across databases. But why don't you see that pattern much any more?
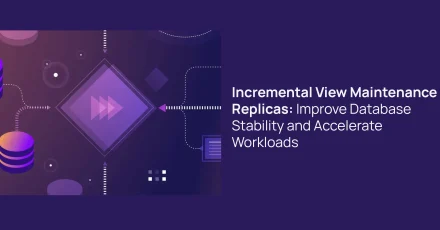
Aug 14, 2024
Incremental View Maintenance Replicas: Improve Database Stability and Accelerate Workloads
IVMRs can deliver 1000x performance for read-heavy workloads, without losing freshness, and do so at a fraction of the price of a traditional replica.
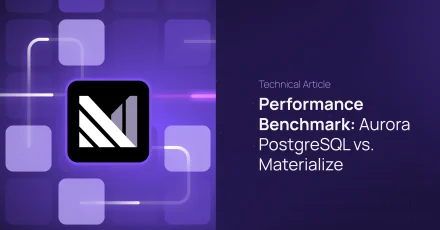
Aug 12, 2024
Performance Benchmark: Aurora PostgreSQL vs. Materialize
Materialize outperforms Aurora for complex queries over relatively small data volumes. Here are the benchmarks.
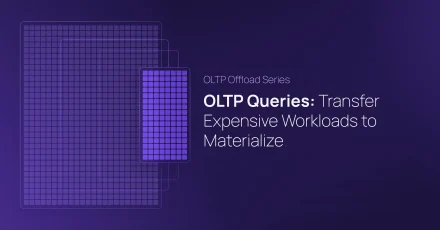
Aug 1, 2024
OLTP Queries: Transfer Expensive Workloads to Materialize
There are many different methods for OLTP offload, and in the following blog, we will examine the most popular options.
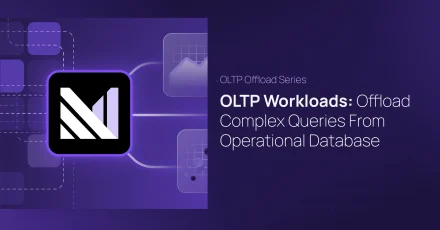
Jul 23, 2024
OLTP Workloads: Offload Complex Queries From Your Operational Database
Read the following blog to learn about OLTP vs. OLAP, problems with complex OLTP workloads, and the case for OLTP offload.
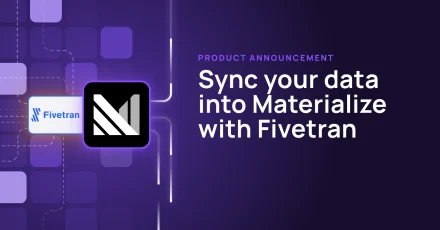
Jul 22, 2024
Sync your data into Materialize with Fivetran
A breakdown of how we built the Materialize Fivetran Destination with Fivetran's Partner SDK, and how this unlocks new workflows in Materialize.
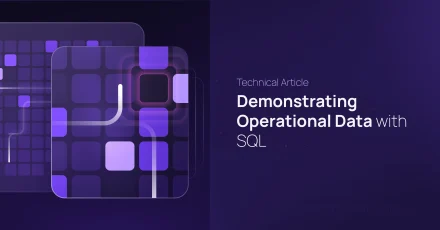
Jul 17, 2024
Demonstrating Operational Data with SQL
In this post, we'll build a recipe for a generic live data source using standard SQL primitives and some Materialize magic.
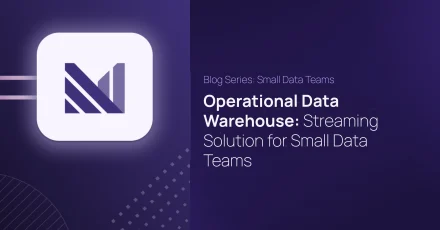
Jul 10, 2024
Operational Data Warehouse: Streaming Solution for Small Data Teams
Under-resourced small data teams can now leverage a SaaS solution with streaming data and SQL support to build real-time applications.
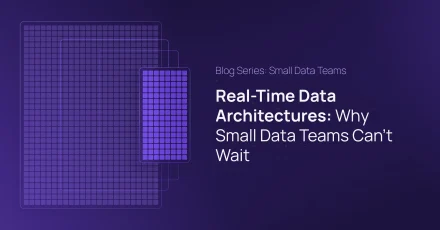
Jul 2, 2024
Real-Time Data Architectures: Why Small Data Teams Can't Wait
Small data teams can't wait to build real-time data architectures. Find out why, and how they're approaching the problem.
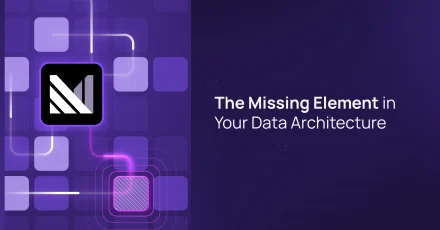
Jun 26, 2024
The Missing Element in Your Data Architecture
Learn how replacing the legacy materialized view with a new element is transformational for your data stack.
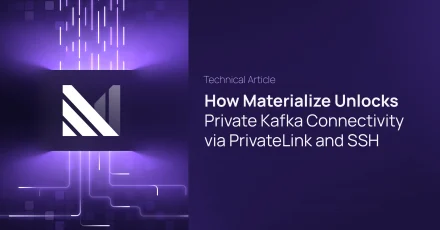
Jun 10, 2024
How Materialize Unlocks Private Kafka Connectivity via PrivateLink and SSH
Here's how we developed frictionless private networking for Kafka by using librdkafka.
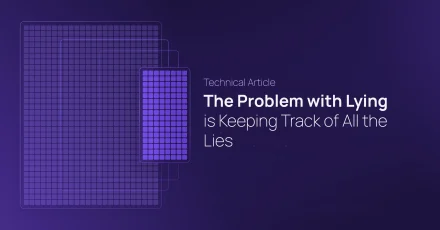
Jun 5, 2024
The Problem with Lying is Keeping Track of All the Lies
Or why clear consistency guarantees are how to stay sane when programming distributed systems.
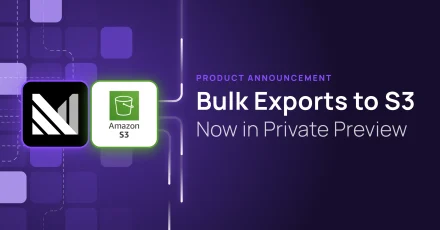
Jun 3, 2024
Bulk exports to S3, now in Private Preview!
Export a snapshot of your data to Amazon S3 object storage as an intermediary to sink data to a broader set of systems downstream
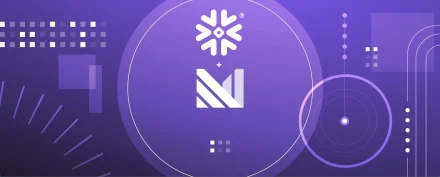
May 30, 2024
Celebrating our newest partnership at Data Cloud Summit
Materialize is now partners with Snowflake. Celebrate with us next week at Snowflake Data Cloud Summit.
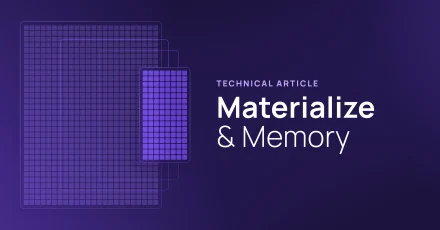
May 16, 2024
Materialize and Memory
We reduced memory requirements for many users by nearly 2x, resulting in significant cost-savings.
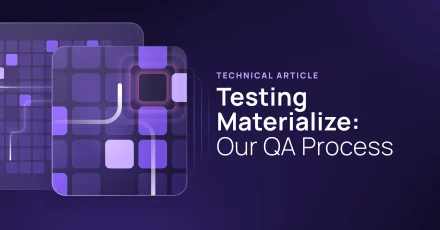
May 13, 2024
Testing Materialize: Our QA Process
The following blog will show you we keep our customers and developers happy with our rigorous QA process, including our tools and testing methods.
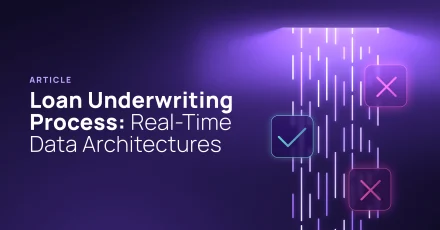
May 8, 2024
Loan Underwriting: Real-Time Data Architectures
This blog will provide an overview of the different data architectures lenders use to power real-time loan underwriting.
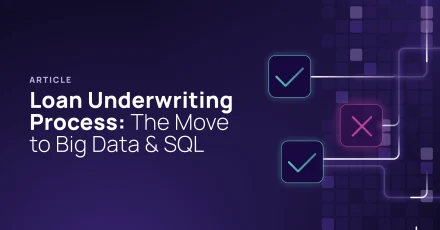
May 7, 2024
Loan Underwriting Process: The Move to Big Data & SQL
In this blog, we'll examine the loan underwriting process, including the current landscape, credit modeling, and the move toward big data and SQL.
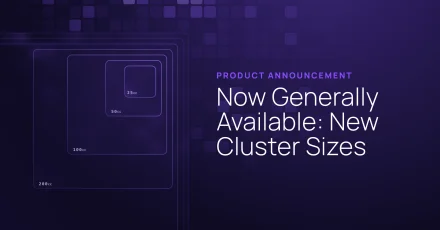
May 1, 2024
Now Generally Available: New Cluster Sizes
New names, new sizes, plus spill-to-disk capabilities
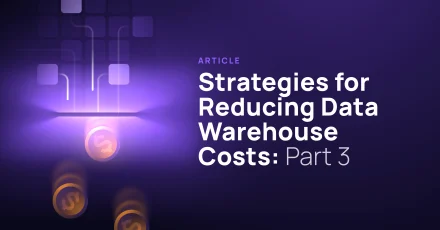
Apr 16, 2024
Strategies for Reducing Data Warehouse Costs: Part 3
Decrease your data warehouse costs by sinking precomputed results and leveraging real-time analytics.
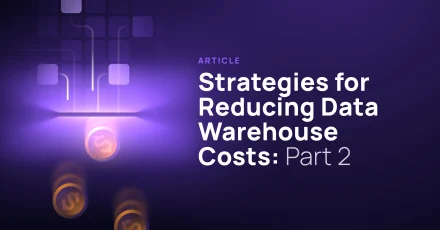
Apr 16, 2024
Strategies for Reducing Data Warehouse Costs: Part 2
Here's how to save money on your data warehouse bill with normalized data models and data mesh principles.
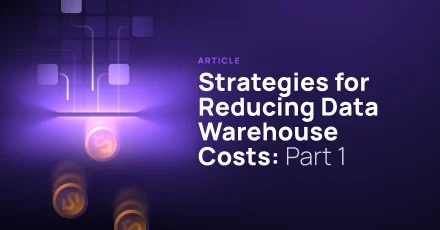
Apr 9, 2024
Strategies for Reducing Data Warehouse Costs: Part 1
With Materialize, teams can lower the cost of their data warehouse bill and implement new use cases.
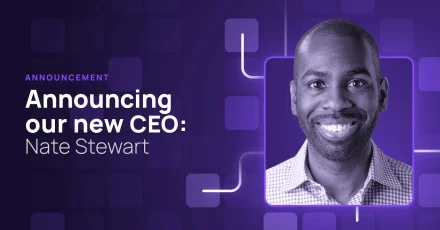
Apr 8, 2024
Announcing our new CEO: Nate Stewart
Materialize welcomes new CEO Nate Stewart, who previously served on the Materialize board and comes to us from Cockroach Labs.
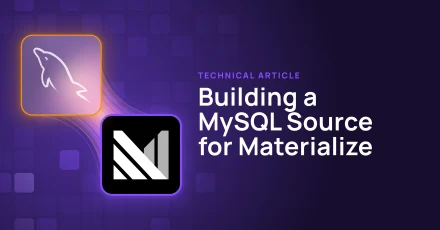
Mar 21, 2024
Building a MySQL source for Materialize
An in-depth breakdown of how we architected and built a native MySQL CDC source
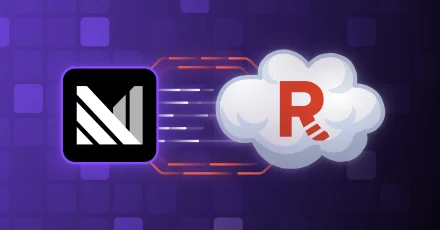
Mar 19, 2024
Materialize + Redpanda Serverless: Simplified developer experience for real-time apps
Combining Redpanda Serverless with Materialize makes developing streaming data apps easier than ever before.
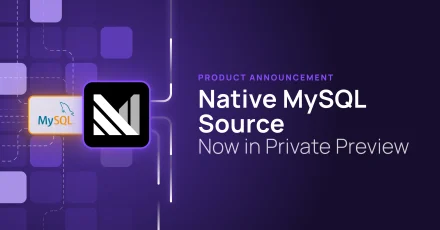
Mar 15, 2024
Native MySQL Source, now in Private Preview
Access the freshest data in MySQL to power your operational workflows
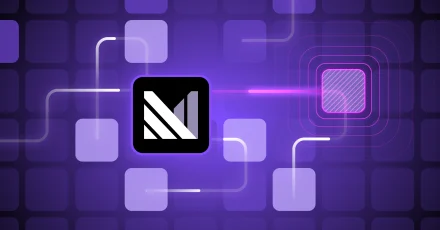
Mar 7, 2024
Real-Time Fraud Detection: Analytical vs. Operational Data Warehouses
In this blog, we’ll explain the different roles of analytical and operational data warehouses in building real-time fraud detection systems.
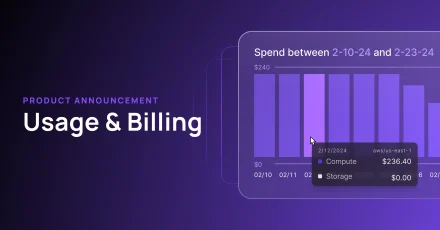
Mar 5, 2024
View your usage and billing history
Get complete visibility into your usage trends and billing history to manage your spend effectively
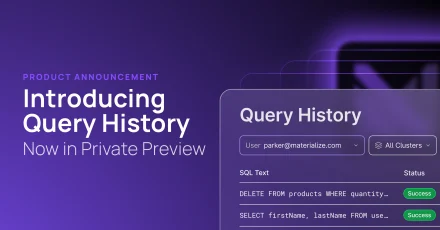
Feb 29, 2024
Introducing Query History
Now in Private Preview, Query History lets you monitor your SQL query performance to detect potential bottlenecks
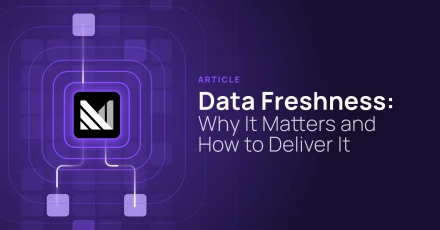
Feb 23, 2024
Data Freshness: Why It Matters and How to Deliver It
Data freshness is essential for real-time business use cases. Here's how an operational data warehouse powers your business processes with fresh data.
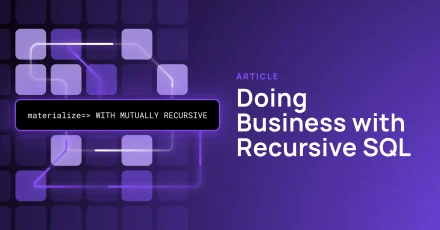
Feb 12, 2024
Doing business with recursive SQL
Learn how recursive SQL provides an elegant solution for a fundamental use case in economics - stable matching.
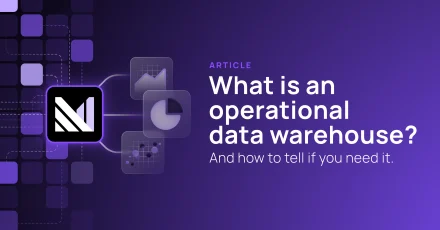
Feb 2, 2024
What is an operational data warehouse?
Learn how an operational data warehouse enables organizations to use their freshest data for day-to-day decision-making
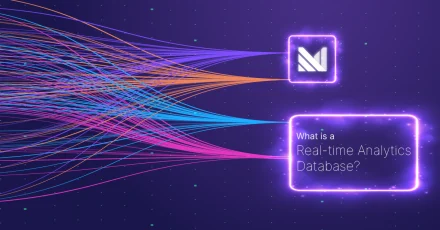
Jan 26, 2024
What is a real-time analytics database?
Discover the essentials of real-time analytics databases, their benefits, and how they compare to traditional databases for better operational decision-making.
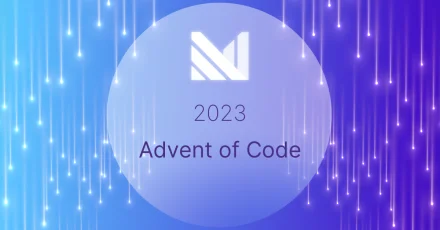
Jan 19, 2024
Materialize and Advent of Code: Using SQL to solve your puzzles!
The Materialize team participated in Advent of Code 2023 and took a bold approach in using SQL to solve each puzzle. Check it out.
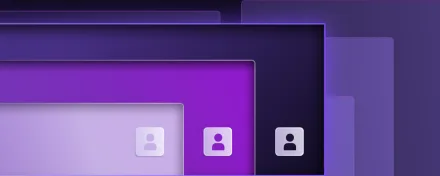
Jan 11, 2024
Responsiveness and Operational Agility
See how Materialize supports operational work with responsiveness.
Dec 21, 2023
How we built the SQL Shell
Learn how we built an in-browser SQL shell that empowers Materialize users to interact with their databases
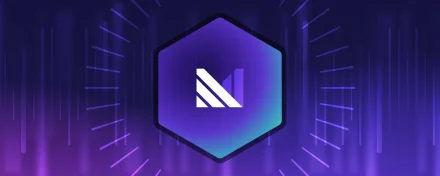
Oct 27, 2023
Compile Times and Code Graphs
Recently, I've felt the pain of long Rust compile times at Materialize, and so was motived to improve them a bit. Here's how I did it.
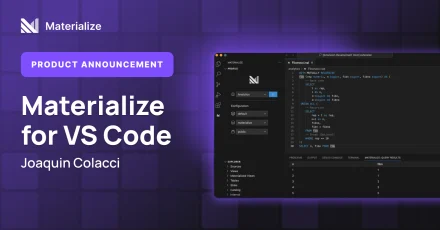
Oct 16, 2023
VS Code Integration Guide | Materialize
Integrate Materialize with VS Code for schema exploration, SQL validation & query execution, all within your IDE for efficient development.
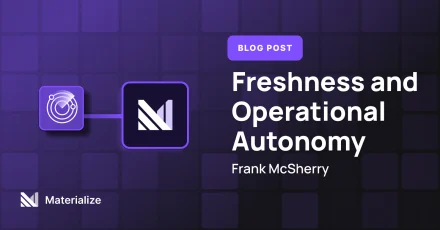
Oct 12, 2023
Freshness and Operational Autonomy
At the heart of freshness in Materialize is autonomous proactive work, done in response to the arrival of data rather than waiting for a user command.
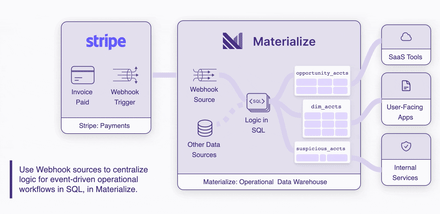
Sep 28, 2023
Announcing Webhook Sources
Today Materialize customers can create webhook sources, making it much easier to pipe in events from a long tail of SaaS platforms, services, and tools.
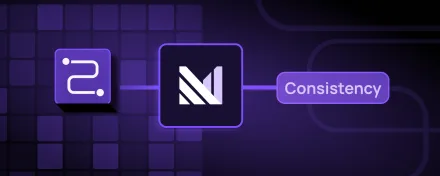
Sep 26, 2023
Consistency and Operational Confidence
Materialize's consistency guarantees are key for confidence in data warehouses. Understand the benefits & see real-world tests in action.
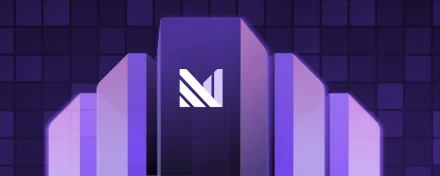
Sep 22, 2023
A guided tour through Materialize's product principles
Take a guided tour through Materialize's three pillars of product value, and see how we think about providing value for your operational workloads.
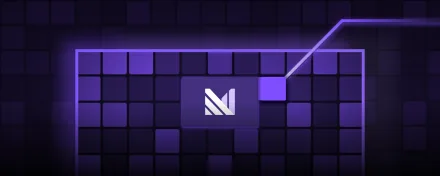
Sep 12, 2023
Operational Data Warehouse Overview | Materialize
We've built Materialize as a new kind of data warehouse, optimized to handle operational data work with the same familiar process from analytical warehouses.
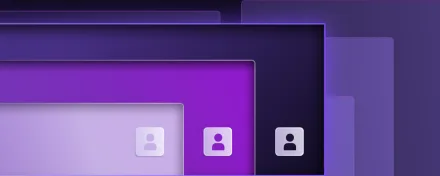
Aug 31, 2023
RBAC now available for all customers
Comprehensive RBAC for Materialize users ensures secure, production-grade environment management & access control.
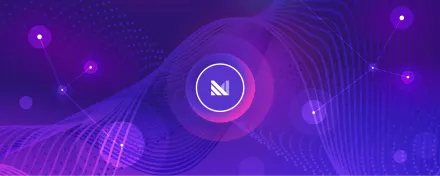
Aug 29, 2023
Lower Data Freshness Costs for Teams | Materialize
Materialize has a subtly different cost model that is a huge advantage for operational workloads that need fresh data.
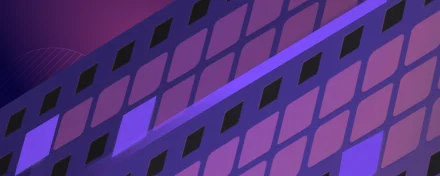
Aug 1, 2023
Capturing Change Data Capture (CDC) Data
An illustration of the unexpectedly high downstream cost of clever optimizations to change data capture.
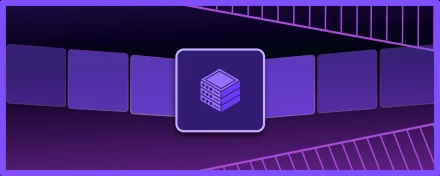
Jul 27, 2023
Cloud Data Warehouse Uses & Misuses | Materialize
Data Warehouses are great for many things but often misused for operational workloads.
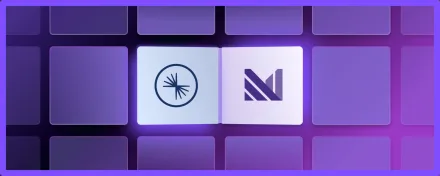
Jul 18, 2023
Confluent & Materialize Expand Streaming | Materialize
Materialize & Confluent partnership offers SQL on Kafka capabilities for efficient data team integration.
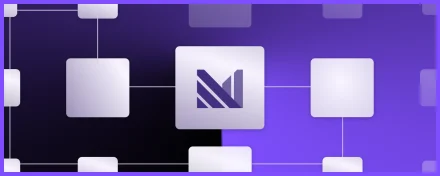
Jul 12, 2023
Recursive SQL Queries in Materialize | Materialize
Support for recursive SQL queries in Materialize is now available.
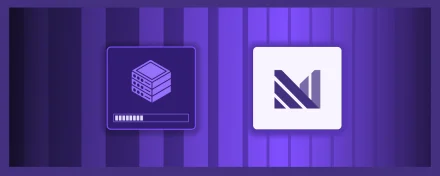
Jun 2, 2023
Shifting Workloads from Data Warehouses | Materialize
A framework for understanding why and when to shift a workload from traditional cloud data warehouses to Materialize.
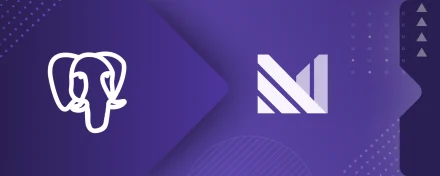
May 18, 2023
Real-Time Postgres Views Updates | Materialize
Major updates to PostgreSQL streaming replication allow for real-time & incrementally updated materialized views with Materialize.
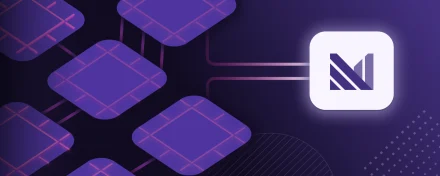
May 11, 2023
When to use Materialize vs a Stream Processor
If you're already familiar with stream processors you may wonder: When is it better to use Materialize vs a Stream Processor? And why?
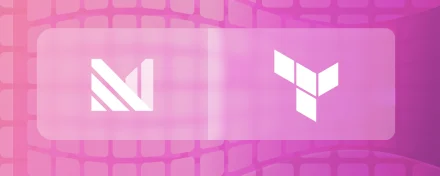
Apr 25, 2023
A Terraform Provider for Materialize
Materialize maintains an official Terraform Provider you can use to manage your clusters, replicas, connections and secrets as code.
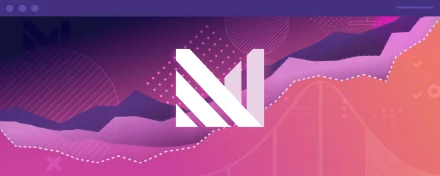
Apr 20, 2023
Everything you need to know to be a Materialize power-user
Master Materialize for enhanced scale, performance & power with key internal insights. A guide for aspiring power-users.
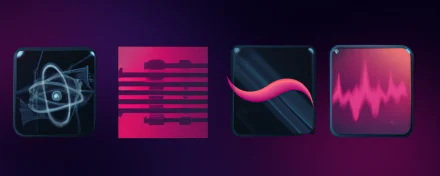
Apr 5, 2023
The Four ACID Questions
Four questions, and their answers, to explain ACID transactions and how they are handled within Materialize.
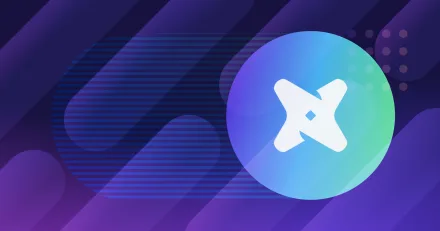
Mar 9, 2023
Towards Real-Time dbt
Explore strategies for unleashing real-time dbt, from materializing views to leveraging micro-batches and incrementally maintained views.
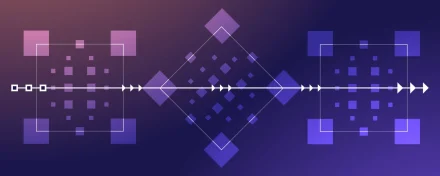
Feb 23, 2023
The Software Architecture of Materialize
Materialize aims to be usable by anyone who knows SQL, but for those interested in going deeper and understanding the architecture powering Materialize, this post is for you!
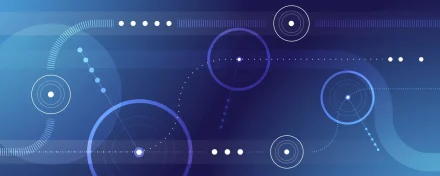
Feb 16, 2023
When to Use Indexes and Materialized Views
If you are familiar with materialized views and indexes from other databases, this article will help you apply that understanding to Materialize.
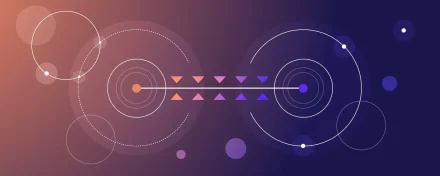
Feb 9, 2023
Building Differential Dataflow from Scratch
Let's build (in Python) the Differential Dataflow framework at the heart of Materialize, and explain what it's doing along the way.
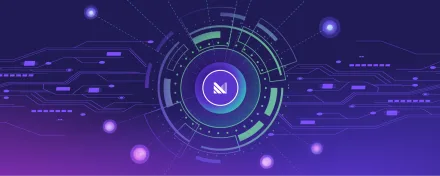
Jan 31, 2023
Clusters, explained with Data Warehouses
If you're familiar with data warehouses, this article will help you understand Materialize Clusters in relation to well-known components in Snowflake.
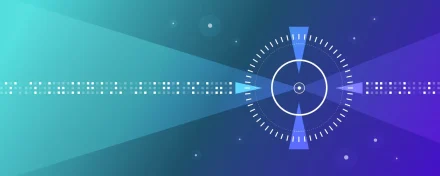
Jan 18, 2023
Delta Joins and Late Materialization
Understand how to optimize joins with indexes and late materialization.
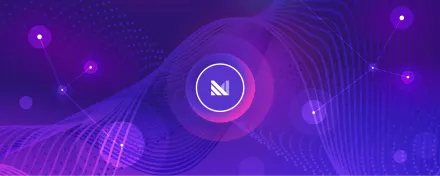
Jan 11, 2023
Recursion in Materialize
Understanding recursion in Materialize & its significance in differential dataflow for SQL updates.
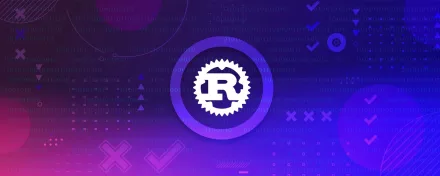
Dec 6, 2022
Rust for high-performance concurrency and network services
Materialize is written in Rust. Why did we make that decision and how has it turned out for the project?
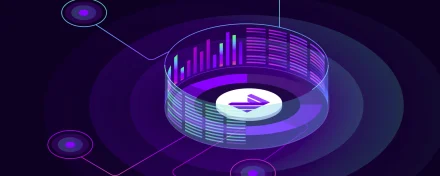
Oct 19, 2022
Real-Time Customer Data Platform Views on Materialize
Let's demonstrate the unique features of Materialize by building the core functionality of a customer data platform.
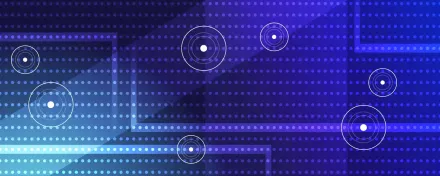
Oct 18, 2022
How and why is Materialize compatible with PostgreSQL?
As an operational data store, Materialize is fundamentally different on the inside, but it's compatible with PostgreSQL in a few important ways.
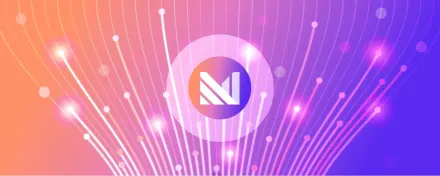
Oct 3, 2022
Announcing the next generation of Materialize
Today, we’re excited to announce a product that we feel is transformational: a persistent, scalable, cloud-native Materialize.
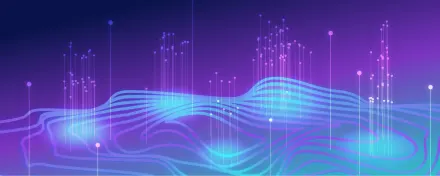
Jul 27, 2022
Indexes: A Silent Frenemy
Insights on how indexes impact scaling in databases & their evolution in streaming-first data warehouses.
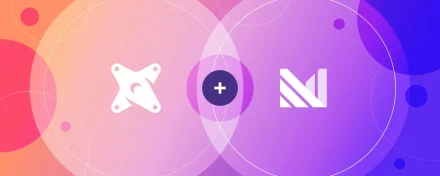
Jul 14, 2022
Real-time data quality tests using dbt and Materialize
Real-time SQL monitoring & data quality tests with dbt & Materialize for continuous insights as data evolves.
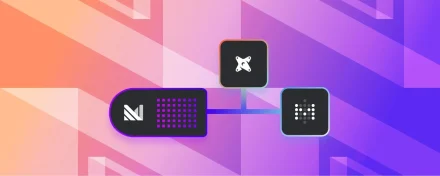
Jun 15, 2022
Managing streaming analytics pipelines with dbt
Using dbt to manage and document a streaming analytics workflow from a message broker to Metabase.
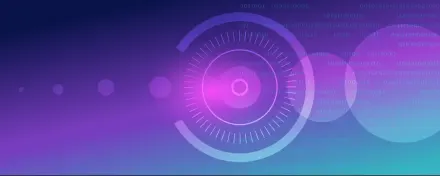
Jun 14, 2022
Virtual Time for Scalable Performance | Materialize
The key to Materialize's ability to separate compute from storage and scale horizontally without sacrificing consistency is a concept called virtual time.
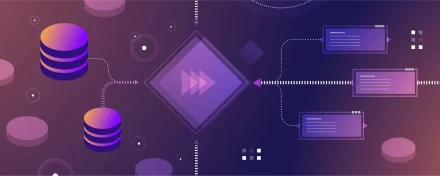
Jun 9, 2022
Let’s talk about Data Apps
What is a Data Application? How do they help our customers? What new challenges do we face when building Data Apps? Here's our perspective.
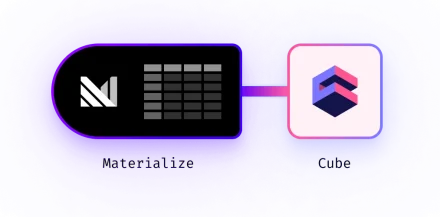
May 13, 2022
Announcing the Materialize Integration with Cube
Connect headless BI tool Cube.js to the read-side of Materialize to get Rest/GraphQL API's, Authentication, metrics modelling, and more out of the box.
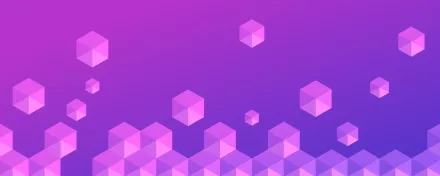
May 6, 2022
Materialize's unbundled cloud architecture
Materialize's new cloud architecture enhances scalability & performance by breaking the materialized
binary into separate services.
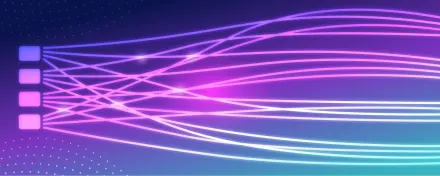
Apr 25, 2022
Creating a Real-Time Feature Store with Materialize
Materialize provides a real-time feature store that updates dimensions with new data instantly & maintains speed & accuracy.
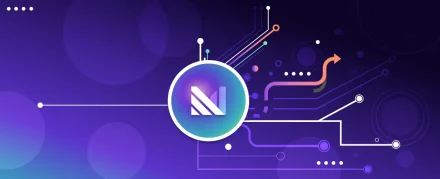
Mar 3, 2022
Subscribe to changes in a view with Materialize
Real-time SQL query & view update subscriptions are made simple with Materialize's SUBSCRIBE feature.
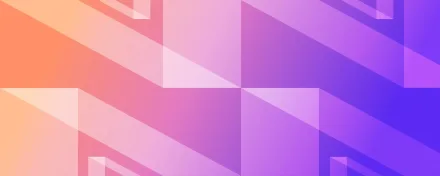
Mar 1, 2022
What's new in Materialize? Vol. 2
Comprehensive updates in Materialize Vol. 2: AWS roles, PostgreSQL enhancements, Schema Registry SSL, & more for streamlined data management.
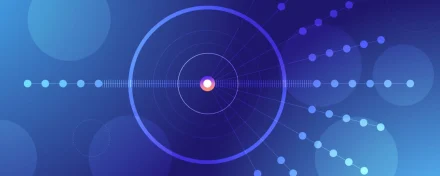
Feb 16, 2022
Direct PostgreSQL Replication Stream Setup | Materialize
Comprehensive guide on using PostgreSQL's write-ahead log as a data source for Materialize, with technical insights & benefits.
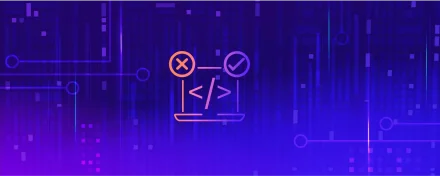
Feb 1, 2022
Taming the beast that is a SQL database
In this article, we will talk about one of the ways we approach the testing of the SQL engine of the product at Materialize. We hope to cover other modules and interesting angles in the future.
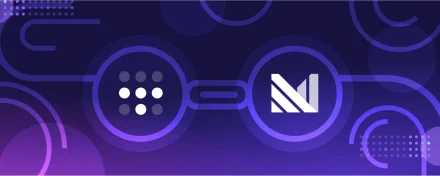
Jan 19, 2022
Introducing: Tailscale + Materialize
Materialize Cloud integrates with Tailscale, offering secure & easy connection of clusters to private networks using WireGuard protocol.
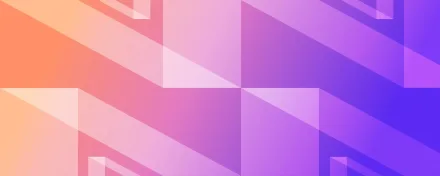
Dec 20, 2021
What's new in Materialize? Volume 1
Stay updated with Materialize: Kafka source metadata, protobuf & schema registry integration, time bucketing, Metabase, cloud metrics & monitoring enhancements.
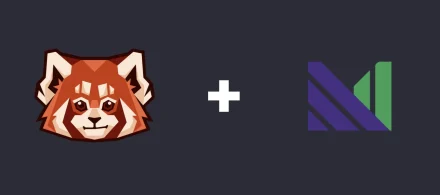
Oct 19, 2021
Stream Analytics with Redpanda & Materialize | Materialize
Enhance your data workflows with Redpanda & Materialize for faster & more efficient streaming analytics. Get insights on integration & usage.
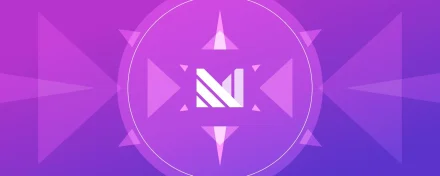
Sep 30, 2021
Materialize Secures $60M Series C Funding | Materialize
Materialize raises another round of funding to help build a cloud-native streaming data warehouse.
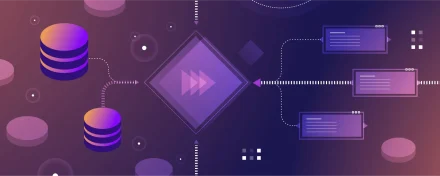
Sep 21, 2021
Change Data Capture is having a moment. Why?
Change Data Capture (CDC) is finally gaining widespread adoption as a architectural primitive. Why now?
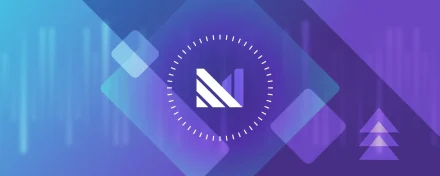
Sep 13, 2021
Materialize Cloud Enters Open Beta
Materialize Cloud, now in open beta, offers real-time data warehousing for immediate insights & action on live data.
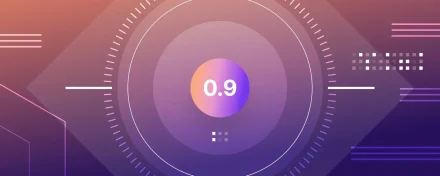
Aug 27, 2021
Release: 0.9
Materialize's Release 0.9 introduces an Operational Data Warehouse optimized for real-time data actions & cloud efficiency.
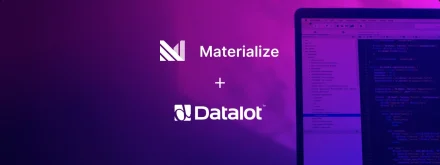
Aug 5, 2021
Materialize & Datalot: Real-time Application Development
Materialize & Datalot collaborate on cutting-edge real-time application development, leveraging streaming data for immediate insights & action.
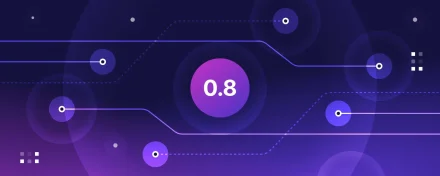
Jun 14, 2021
Release: 0.8
Comprehensive insights & updates on Materialize's Release 0.8, enhancing real-time data warehousing capabilities for immediate action.
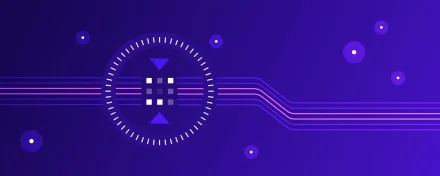
Jun 2, 2021
Maintaining Joins using Few Resources
Efficiently maintain joins with shared arrangements & reduce resource usage with Materialize's innovative approach.
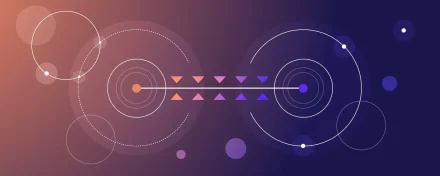
Apr 29, 2021
Generalizing linear operators in differential dataflow
Differential dataflow uses simple linear operators: map
, filter
, flat_map
and complex: explode
and temporal filter operators. But, with some thinking, we can generalize them all to a restricted form of join.
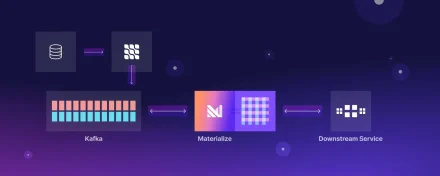
Apr 27, 2021
Join Kafka with a Database using Debezium and Materialize
Debezium and Materialize can be used as powerful tools for joining high-volume streams of data from Kafka and tables from databases.
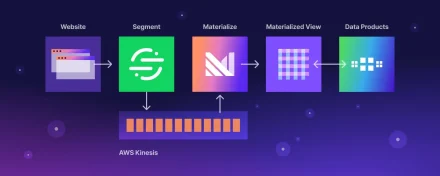
Apr 21, 2021
Real-time A/B Testing with Segment & Kinesis | Materialize
Build a real-time A/B testing stack with Segment, Kinesis and Materialize.
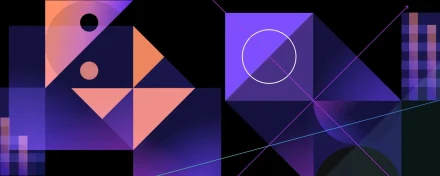
Mar 24, 2021
dbt & Materialize: Streamline Jaffle Shop Demo | Materialize
Let's demonstrate how to manage streaming SQL in Materialize with dbt by porting the classic dbt jaffle-shop demo scenario to the world of streaming.
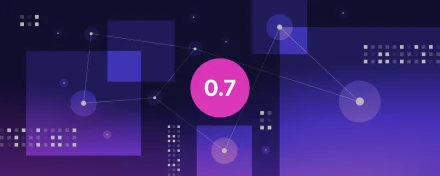
Mar 9, 2021
Release: 0.7
Comprehensive insights & updates in Materialize's Release 0.7, enhancing real-time data warehouse capabilities.
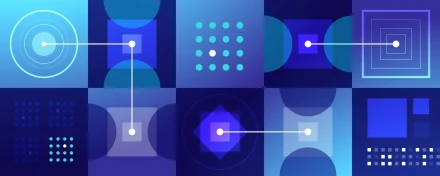
Mar 1, 2021
How Materialize and other databases optimize SQL subqueries
Insight into SQL subquery optimization & how Materialize's approach differs from other databases, enhancing query performance.
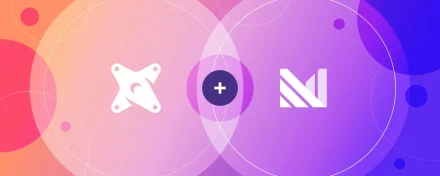
Mar 1, 2021
Introducing: dbt + Materialize
Efficient SQL data transformations & real-time analytics with dbt + Materialize: a powerful operational data warehouse combo.
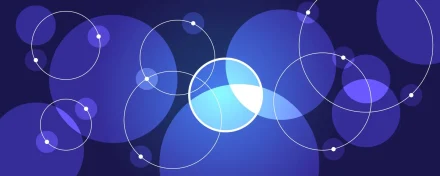
Feb 16, 2021
Temporal Filters: Enabling Windowed Queries in Materialize
Temporal filters give you a powerful SQL primitive for defining time-windowed computations over temporal data.
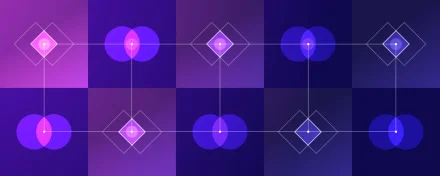
Jan 20, 2021
Efficient Real-Time App with TAIL | Materialize
Let's build a python application to demonstrate how developers can create real-time, event-driven experiences for their users, powered by Materialize.
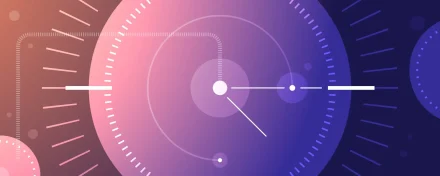
Jan 14, 2021
Slicing up Temporal Aggregates in Materialize
Comprehensive guide on slicing temporal aggregates with Materialize for real-time data analysis & actionable insights.
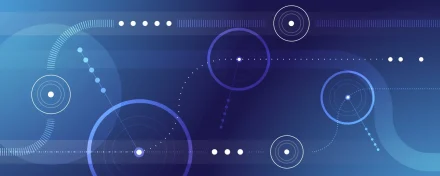
Jan 11, 2021
Understanding Differential Dataflow
How to write algorithms in differential dataflow, using Conway's Game of Life as an example.
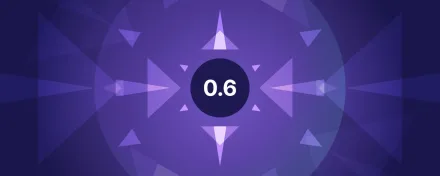
Jan 7, 2021
Release: 0.6
Materialize's Release 0.6 enhances cloud data warehousing with real-time streaming capabilities for immediate action on live data.
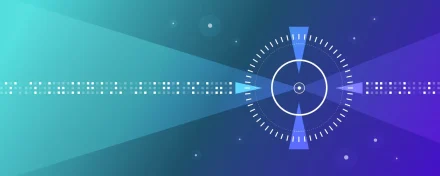
Dec 14, 2020
Joins in Materialize
Comprehensive guide to implementing joins in Materialize, covering binary to delta joins for efficient streaming systems.
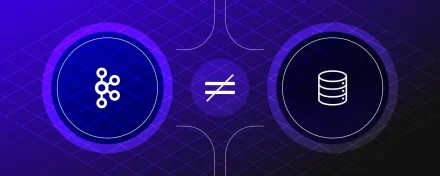
Dec 8, 2020
Kafka is not a Database
In principle, it is possible to use Kafka as a database. But in doing so you will confront every hard problem that database management systems have faced for decades
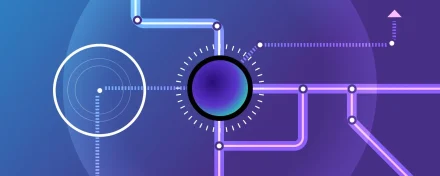
Dec 2, 2020
Live Maintained Views on Boston Transit to Run at Home
Real-time apps for Boston Transit with live data are easy to set up using Materialize; see two examples you can run at home.
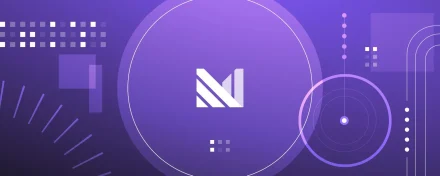
Nov 30, 2020
Materialize Raises a Series B
Materialize secures Series B funding to enhance its Operational Data Warehouse with real-time streaming capabilities for immediate data action.
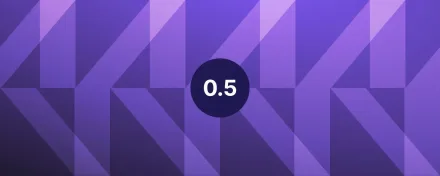
Nov 24, 2020
Release: Materialize 0.5
Materialize 0.5 operational data warehouse offers real-time action on live data for efficient & immediate insights.
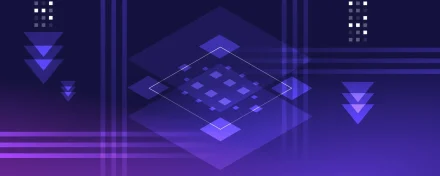
Sep 30, 2020
Materialize under the Hood
An in-depth look at Materialize, the Operational Data Warehouse with streaming capabilities for real-time data action.
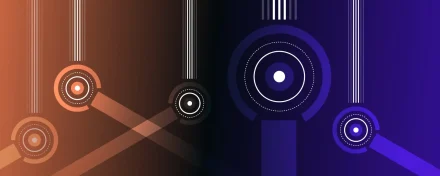
Aug 18, 2020
Lateral Joins and Demand-Driven Queries
Comprehensive guide to using Materialize's LATERAL
join for efficient query patterns in incremental view maintenance engines.
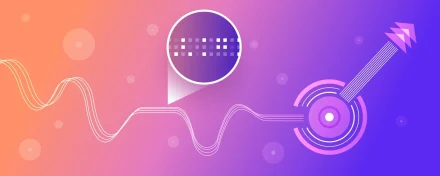
Aug 13, 2020
Change Data Capture (part 1)
Here we set the context for and propose a change data capture protocol: a means of writing down and reading back changes to data.
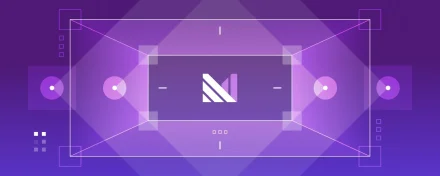
Aug 11, 2020
Why, How, and When To Use Materialized Views
Discover how to reduce database query costs with Materialized Views. This guide will walk you through the benefits, creation process, and impact on database efficiency.
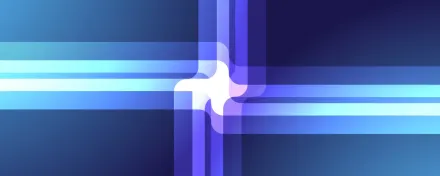
Aug 6, 2020
Why not RocksDB for streaming storage?
An explanation of our rationale for why Materialize chose not to use RocksDB as its underlying storage engine.
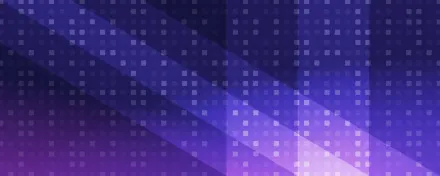
Aug 4, 2020
Robust Reductions in Materialize
Comprehensive guide to implementing robust reductions in Materialize, ensuring efficient & real-time data processing.
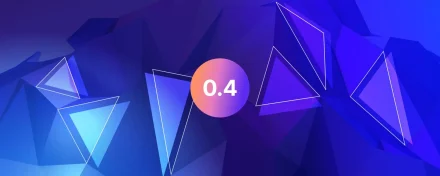
Jul 28, 2020
Release: Materialize 0.4
Materialize 0.4 introduces an Operational Data Warehouse with real-time streaming capabilities for immediate data action & analysis.
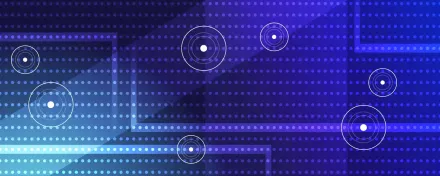
Jul 24, 2020
Streaming TAIL to the Browser - A One Day Project
Real-time data streaming directly to your browser with Materialize's latest one-day project; understand the technical journey & outcomes.
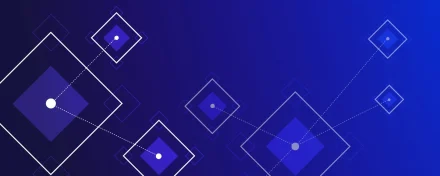
Jul 14, 2020
Eventual Consistency isn't for Streaming
Understand why eventual consistency isn't suitable for streaming systems & the systematic errors it can cause with Materialize's insights.
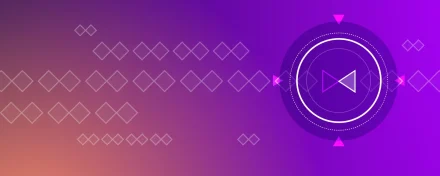
Jun 22, 2020
Rust for Data-Intensive Computation
Harness the power of Rust for data-intensive tasks with Materialize, offering real-time insights & performance benefits.
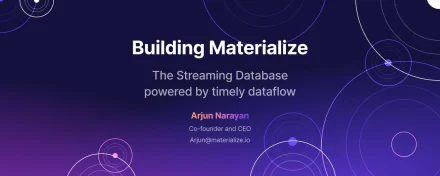
Jun 8, 2020
CMU DB Talk: Building Materialize
Arjun Narayan introduces the CMU DB group to streaming databases, the problems they solve, and specific architectural decisions in Materialize.
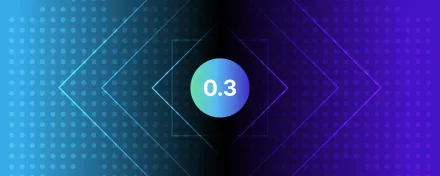
Jun 1, 2020
Release: Materialize 0.3
Materialize 0.3, an Operational Data Warehouse with cloud & streaming capabilities, optimizes real-time data action.
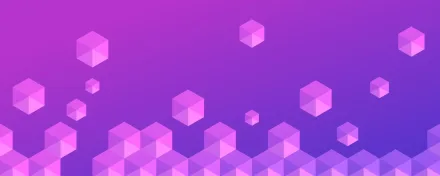
May 5, 2020
Managing memory with differential dataflow
Insights on how Differential Dataflow manages & limits memory use for processing unbounded data streams, ensuring efficiency.
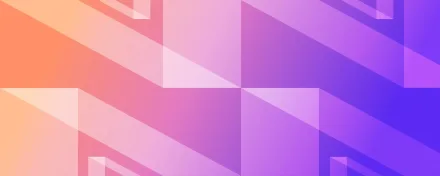
Mar 31, 2020
Consistency Guarantees in Data Streaming | Materialize
Understand the necessary consistency guarantees for a streaming data platform & how they ensure accurate data views.
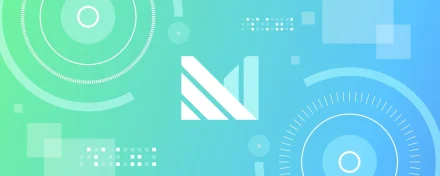
Mar 27, 2020
Upserts in Differential Dataflow
Comprehensive guide to implementing upserts in differential dataflow with Materialize for real-time data warehouse optimization & efficiency.
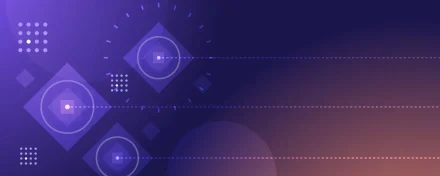
Mar 18, 2020
Taking Materialize for a spin on NYC taxi data
Experience real-time data analysis with Materialize on NYC taxi data, showcasing a practical application of streaming SQL.
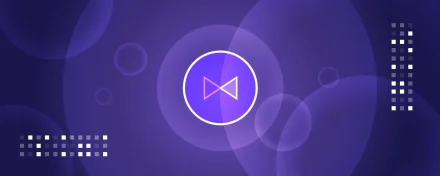
Feb 24, 2020
View Maintenance: A New Approach to Data Processing
Materialize's approach to data processing & view maintenance offers real-time insights for immediate action on live data.
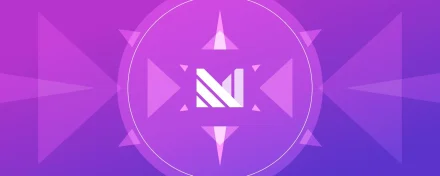
Feb 20, 2020
Materialize Beta: The Details
Materialize Beta offers insights on a cloud data warehouse with real-time streaming capabilities for immediate action on current data.
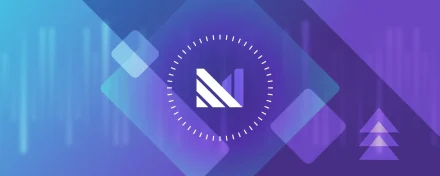
Feb 18, 2020
Introducing Materialize: the Streaming Data Warehouse
Materialize offers a streaming data warehouse for real-time analytics & interoperability with millisecond latency, revolutionizing data handling.